The Performance of Machine Learning for Chronic Kidney Disease Diagnosis
DOI:
https://doi.org/10.46604/emsi.2023.11285Keywords:
chronic kidney disease, machine learning, performance, scoping reviewAbstract
This paper aims to review the performance of different machine learning (ML) models and develop models for the automated diagnosis of chronic kidney disease. To detect chronic kidney disease with better precision, selecting the right and better-performing ML model is significant as it improves the precision and accuracy of the chronic kidney disease diagnosis. The study uses the Joana Briggs Institute (JBI) scoping review methodology, which involves different steps such as searching relevant literature, conducting the review, and reporting the review result. In the search, the year of publication and the indexing of journals where the studies are published is used as inclusion and exclusion criteria. The review result shows that the current chronic kidney disease detection has focused on the development of ensemble-based and deep-learning methods. The deep learning method can achieve a higher accuracy of 99.75%.
References
V. Shanmugarajeshwari and M. Ilayaraja, “Chronic Kidney Disease for Collaborative Healthcare Data Analytics Using Random Forest Classification Algorithms,” International Conference on Computer Communication and Informatics (ICCCI), pp. 1-14, January 2021.
Y. Amirgaliyev, S. Shamiluulu, and A. Serek, “Analysis of Chronic Kidney Disease Dataset by Applying Machine Learning Methods,” IEEE 12th International Conference on Application of Information and Communication Technologies (AICT), pp. 1-4, October 2018.
Z. Saringat, A. Mustapha, R. R. Saedudin, and N. A. Samsudin, “Comparative Analysis of Classification Algorithms for Chronic Kidney Disease Diagnosis,” Bulletin of Electrical Engineering and Informatics, vol. 8, no. 4, pp. 1496-1501, December 2019.
K. B. Chimwayi, N. Haris, R. D. Caytiles, and N. C. S. Iyengar, “Risk Level Prediction of Chronic Kidney Disease Using Neuro-Fuzzy and Hierarchical Clustering Algorithm (s),” International Journal of Multimedia and Ubiquitous Engineering, vol. 12, no. 8, pp. 23-36, 2017.
M. Bhatt and T. Kasbe, “A Survey on Chronic Kidney Disease Diagnosis Using Fuzzy Logic,” IEEE International Symposium on Smart Electronic Systems (iSES) (Formerly iNiS), pp. 252-256, December 2019.
S. Sharma, V. Sharma, and A. Sharma, “A Two Stage Hybrid Ensemble Classifier Based Diagnostic Tool for Chronic Kidney Disease Diagnosis Using Optimally Selected Reduced Feature Set,” International Journal of Intelligent Systems and Applications in Engineering, vol. 6, no. 2, pp. 113-122, April-June 2018.
E. M. Senan, M. H. Al-Adhaileh, F. W. Alsaade, T. H. H. Aldhyani, A. A. Alqarni, N. Alsharif, et al., “Diagnosis of Chronic Kidney Disease Using Effective Classification Algorithms and Recursive Feature Elimination Techniques,” Journal of Healthcare Engineering, vol. 2021, article no. 1004767, June 2021.
G. M. Ifraz, M. H. Rashid, T. Tazin, S. Bourouis, and M. M. Khan, “Comparative Analysis for Prediction of Kidney Disease Using Intelligent Machine Learning Methods,” Computational and Mathematical Methods in Medicine, vol. 2021, article no. 6141470, December 2021.
M. D. J. Peters, C. Marnie, H. Colquhoun, C. M. Garritty, S. Hempel, T. Horsley, et al., “Scoping Reviews: Reinforcing and Advancing the Methodology and Application,” Systematic Reviews, vol. 10, article no. 263, October 2021.
V. Jayalakshmi, L. Nayak, and K. Dharmarajan, “A Survey on Chronic Kidney Disease Detection Using Novel Methods,” International Journal of Pure and Applied Mathematics, vol. 119, no. 10, pp. 125-130, 2018.
K. N. Komal, R. L. Tulasi, and D. Vigneswari, “An Ensemble Multi-Model Technique for Predicting Chronic Kidney Disease,” International Journal of Electrical and Computer Engineering, vol. 9, no. 2, pp. 1321-1326, April 2019.
M. Almasoud and T. E. Ward, “Detection of Chronic Kidney Disease using Machine Learning Algorithms with Least Number of Predictors,” International Journal of Soft Computing and Its Applications, vol. 10, no. 8, pp. 80-96, April 2019.
S. Tekale, P. Shingavi, S. Wandhekar, and A. Chatorikar, “Prediction of Chronic Kidney Disease Using Machine Learning Algorithm,” International Journal of Advanced Research in Computer and Communication Engineering, vol. 7, no. 10, pp. 92-96, October 2018.
U. Pujianto, N. A. Ramadhani, and A. P. Wibawa, “Support Vector Machine with Purified K-Means Clusters for Chronic Kidney Disease Detection,” 2nd East Indonesia Conference on Computer and Information Technology (EIConCIT), pp. 56-60, November 2018.
S. Gupta, V. Bharti, and A. Kumar, “A Survey on Various Machine Learning Algorithms for Disease Prediction,” International Journal of Recent Technology and Engineering, vol. 7, no. 6c, pp. 84-87, 2019.
A.Vijayalakshmi and V. Sumalatha, “Survey on Diagnosis of Chronic Kidney Disease Using Machine Learning Algorithms,” 3rd International Conference on Intelligent Sustainable Systems (ICISS), pp. 590-595, December 2020.
B. Deepika, V. K. R. Rao, D. N. Rampure, P. Prajwal, and D. G. Gowda, “Early Prediction of Chronic Kidney Disease by Using Machine Learning Techniques,” American Journal of Computer Science and Engineering Survey, vol. 8, no. 2, article no. 7, September 2020.
K. M. Almustafa, “Prediction of Chronic Kidney Disease Using Different Classification Algorithms,” Informatics in Medicine Unlocked, vol. 24, article no. 100631, 2021.
O. A. Jongbo, A. O. Adetunmbi, R. B. Ogunrinde, and B. Badeji-Ajisafe, “Development of an Ensemble Approach to Chronic Kidney Disease Diagnosis,” Scientific African, vol. 8, article no. e00456, July 2020.
W. Wang, G. Chakraborty, and B. Chakraborty, “Predicting the Risk of Chronic Kidney Disease (CKD) Using Machine Learning Algorithm,” Applied Sciences, vol. 11, no. 1, article no. 202, December 2020.
T. A. Assegie, “Automated Chronic Kidney Disease Detection Model with Knearest Neighbor,” International Journal of Computer and Information Technology, vol. 10, no. 3, pp. 111-115, May 2021.
C. Mondol, F. M. J. M. Shamrat, Md. R. Hasan, S. Alam, P. Ghosh, Z. Tasnim, et al., “Early Prediction of Chronic Kidney Disease: A Comprehensive Performance Analysis of Deep Learning Models,” Algorithms, vol. 15, no. 9, article no. 308, August 2022.
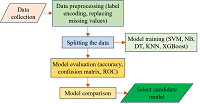
Published
How to Cite
Issue
Section
License
Copyright Notice
Submission of a manuscript implies: that the work described has not been published before that it is not under consideration for publication elsewhere; that if and when the manuscript is accepted for publication. Authors can retain copyright in their articles with no restrictions. Also, author can post the final, peer-reviewed manuscript version (postprint) to any repository or website.
Since Sep. 01, 2023, EMSI will publish new articles with Creative Commons Attribution Non-Commercial License, under Creative Commons Attribution Non-Commercial 4.0 International (CC BY-NC 4.0) License.
The Creative Commons Attribution Non-Commercial (CC-BY-NC) License permits use, distribution and reproduction in any medium, provided the original work is properly cited and is not used for commercial purposes.