Machine Learning for Water Quality Index Forecasting
DOI:
https://doi.org/10.46604/emsi.2024.12870Keywords:
water quality index, machine learning, random forest, support vector machineAbstract
This study aims to forecast water quality in the Tumkur district, Karnataka state, India, to increase pollution levels. Various machine learning techniques, including support vector machines, regression trees, linear regression, and neural networks, are employed. The Water Quality Index (WQI) is determined using parameters such as total hardness, pH, alkalinity, turbidity, chloride, dissolved solids, and conductivity. The dataset is split into training and testing sets (80:20) to assess model performance. Support Vector Machines and Linear Regression outperform other models, achieving R2 values of 0.96 and 0.99 for training and testing, respectively. This research underscores the importance of advanced machine learning techniques for accurate water quality prediction, crucial for effective pollution reduction strategies in the region.
References
F. Rufino, G. Busico, E. Cuoco, T. H. Darrah, and D. Tedesco, “Evaluating The Suitability Of Urban Groundwater Resources for Drinking Water and Irrigation Purposes: An Integrated Approach in The Agro-Aversano Area of Southern Italy,” Environmental Monitoring and Assessment, vol. 191, no. 12, pp. 1-17, November 2019.
R. Mohammadpour, S. Shaharuddin, C. K. Chang, N. A. Zakaria, A. A. Ghani, and N. W. Chan, “Prediction of Water Quality Index in Constructed Wetlands Using Support Vector Machine,” Environmental Science and Pollution Research, vol. 22, no. 2, pp. 6208-6219, November 2014.
T. Tiyasha, T. M. Tung, and Z. M. Yaseen, “A Survey on River Water Quality Modelling Using Artificial Intelligence Models: 2000–2020,” Journal of Hydrology, vol. 585, article no. 124670, June 2020.
N. Sharma, R. Sharma, and N. Jindal, “Machine Learning and Deep Learning Applications-A Vision,” Global Transitions Proceedings, vol. 2, no. 1, pp. 24-28, January 2021.
W. Li, H. Fang, G. Qin, X. Tan, Z. Huang, F. Zeng, et al., “Concentration Estimation of Dissolved Oxygen in Pearl River Basin Using Input Variable Selection and Machine Learning Techniques,” Science of The Total Environment, vol. 731, article no. 139099, August 2020.
V. Sagan, K. T. Peterson, M. Maimaitijiang, P. Sidike, J. Sloan, B. A. Greeling, et al., “Monitoring Inland Water Quality Using Remote Sensing: Potential and Limitations of Spectral Indices, Bio-Optical Simulations, Machine Learning, and Cloud Computing,” Earth-Science Reviews, vol. 205, article no. 103187, June 2020.
Y. Wu, X. Zhang, Y. Xiao, and J. Feng, “Attention Neural Network for Water Image Classification Under IoT Environment,” Applied Sciences, vol. 10, article no. 909, January 2020.
A. R. T. Donders, G. J. M. G. Van Der Heijden, T. Stijnen, and K. G. Moons, “Review: A Gentle Introduction To Imputation of Missing Values,” Journal of Clinical Epidemiology, vol. 59, no. 10, pp. 1087-1091, October 2006.
J. Ma, Y. Ding, J. C. P. Cheng, F. Jiang, and Z. Xu, “Soft Detection of 5-day BOD with Sparse Matrix in City Harbor Water Using Deep Learning Techniques,” Water Research, vol. 170, article no. 115350, March 2020.
A. Š. Tomić, D. Antanasijević, M. Ristić, A. Perić-Grujić, and V. Pocajt, “A Linear and Non-Linear Polynomial Neural Network Modeling of Dissolved Oxygen Content in Surface Water: Inter-and Extrapolation Performance with Inputs' Significance Analysis,” Science of The Total Environment, vol. 610, pp. 1038-1046, January 2018.
M. Zounemat-Kermani, Y. Seo, S. Kim, M. A. Ghorbani, S. Samadianfard, S. Naghshara, et al., “Can Decomposition Approaches Always Enhance Soft Computing Models? Predicting The Dissolved Oxygen Concentration in the St. Johns River, Florida,” Applied Sciences, vol. 9, article no. 2534, June 2019.
K. Chen, H. Chen, C. Zhou, Y. Huang, X. Qi, R. Shen, et al., “Comparative Analysis of Surface Water Quality Prediction Performance and Identification of Key Water Parameters Using Different Machine Learning Models Based on Big Data,” Water Research, vol. 171, article no. 115454, March 2020.
Q. V. Ly, X. C. Nguyen, N. C. Lê, T. D. Truong, T. H. T. Hoang, and T. J. Park, et al., “Application of Machine Learning for Eutrophication Analysis and Algal Bloom Prediction in An Urban River: A 10-year Study of The Han River, South Korea,” Science of The Total Environment, vol. 797, article no. 149040, November 2021.
D. V. V. Prasad, L. Y. Venkataramana, P. S. Kumar, G. Prasannamedha, S. Harshana, and S. J. Srividya, et al., “Analysis And Prediction Of Water Quality Using Deep Learning and Auto Deep Learning Techniques,” Science of The Total Environment, vol. 821, article no. 153311, May 2022.
M. G. Uddin, S. Nash, A. Rahman, and A. I. Olbert, “Performance Analysis of The Water Quality Index Model for Predicting Water State Using Machine Learning Techniques,” Process Safety and Environmental Protection, vol. 169, pp. 808-828, January 2023.
R. C. Karangoda and K. G. N. Nanayakkara, “Use of The Water Quality Index and Multivariate Analysis to Assess Groundwater Quality for Drinking Purpose in Ratnapura District, Sri Lanka,” Groundwater for Sustainable Development, vol. 21, article no. 100910, May 2023.
R. M. Brown, N. I. McClelland, R. A. Deininger, and M. F. O’Connor, A Water Quality Index-Crashing The Psychological Barrier, 1st ed., Boston: Springer, pp. 173-182, 1972.
P. C. Mishra and R. Patel, “Quality of Drinking Water in Rourkela, Outside The Steel Township,” Journal of Environment and Pollution, vol. 8, pp. 165-169, January 2001.
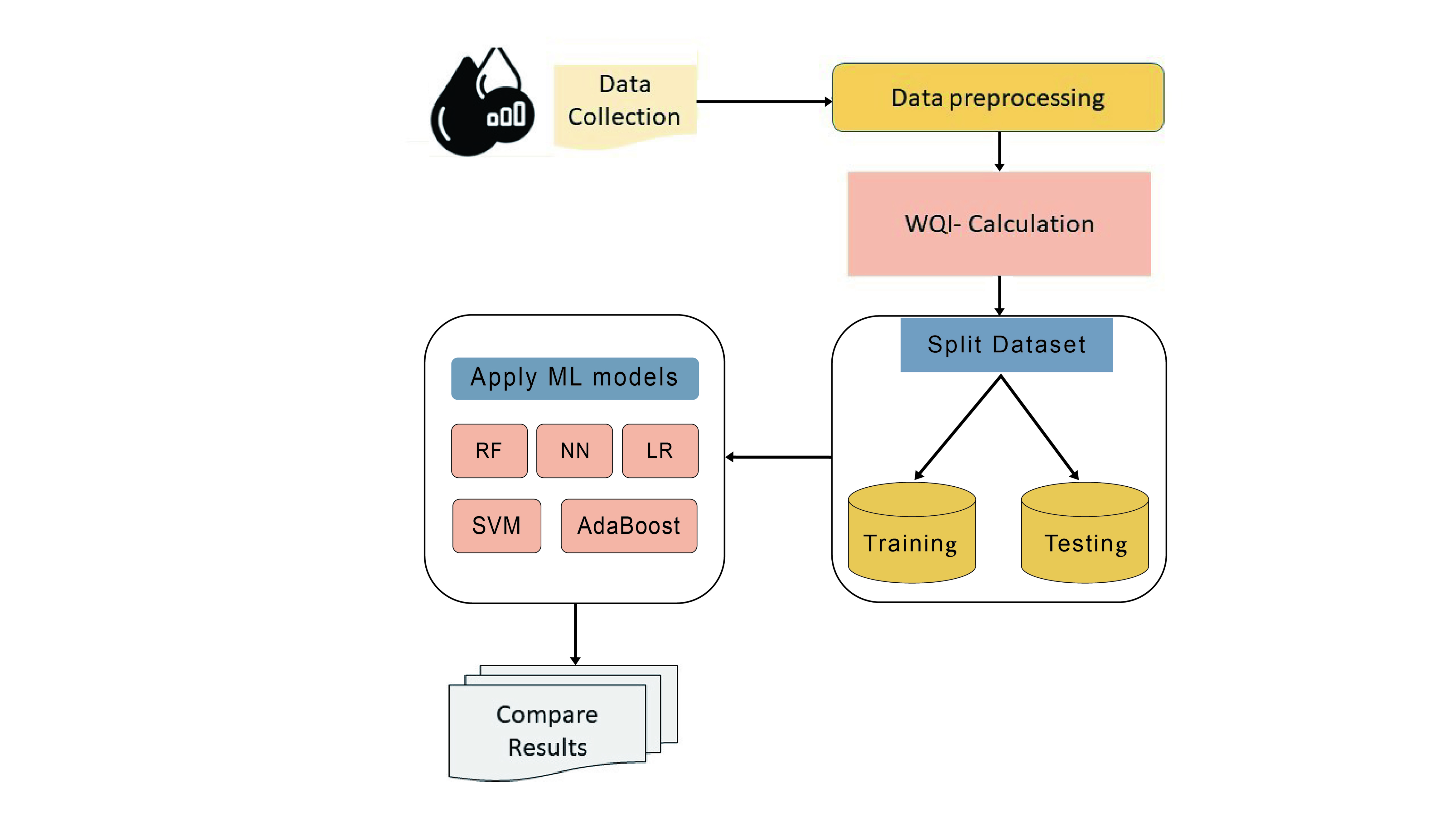
Published
How to Cite
Issue
Section
License
Copyright (c) 2024 Arun Kumar Thimalapur Doddabasappaar, Bilegowdanamane Earappa Yogendra, Prashanth Janardhan, Prema Nisana Siddegowda

This work is licensed under a Creative Commons Attribution-NonCommercial 4.0 International License.
Copyright Notice
Submission of a manuscript implies: that the work described has not been published before that it is not under consideration for publication elsewhere; that if and when the manuscript is accepted for publication. Authors can retain copyright in their articles with no restrictions. Also, author can post the final, peer-reviewed manuscript version (postprint) to any repository or website.
Since Sep. 01, 2023, EMSI will publish new articles with Creative Commons Attribution Non-Commercial License, under Creative Commons Attribution Non-Commercial 4.0 International (CC BY-NC 4.0) License.
The Creative Commons Attribution Non-Commercial (CC-BY-NC) License permits use, distribution and reproduction in any medium, provided the original work is properly cited and is not used for commercial purposes.