Analysis of Association between Caesarean Delivery and Gestational Diabetes Mellitus Using Machine Learning
DOI:
https://doi.org/10.46604/peti.2020.4740Keywords:
C-section, cesarean delivery, GDM, machine learningAbstract
The study aims to analyze the association between gestational diabetes mellitus (GDM) and other risk factors of cesarean delivery using machine learning (ML). The dataset used for the analysis is from the pregnancy risk assessment survey (PRAMS), considered in two scenarios, i.e., all the data is taken, and all the data of the women who developed GDM. Further, the data is developed in two groups Data-I and Data-II by considering multiparous and primiparous women details, respectively. The correlation analysis and major classification algorithms are applied to the data. It is founded that the top risk factors for the first time cesarean delivery are the age, height, weight, race of the women, presence of hypertension and gestational diabetes mellitus. The major risk factor for repeated cesarean delivery is the previous cesarean delivery. The presence of GDM is also one of the risk factors for cesarean delivery.
References
“Reasons For A Cesarean Birth,” https://americanpregnancy.org/labor-and-birth/reasons-for-a-cesarean/, October 13, 2019
P. Chowriappa, S. Dua, and Y. Todorov, Introduction to machine learning in healthcare informatics, Machine Learning in Healthcare Informatics, Springer, 2014, pp. 1-23.
S. Sodsee, “Predicting caesarean section by applying nearest neighbor analysis,” Procedia Computer Science, vol. 31, pp. 5-14, December 2014.
S. Pereira, F. Portela, M. F. Santos, J. Machado, and A. Abelha, “Predicting type of delivery by identification of obstetric risk factors through data mining,” Procedia Computer Science, vol. 64, pp. 601-609, December 2015.
F. Soleimanian, P. Mohammadi, and P. Hakimi, “Application of decision tree algorithm for data mining in healthcare operations: A case study,” International Journal of Computer Applications, vol. 52, no. 6, pp. 21-26, August 2012.
A. Sana, S. Razzaq, and J. Ferzund, “Automated diagnosis and cause analysis of cesarean section using machine learning techniques,” International Journal of Machine Learning and Computing, vol. 2, no. 5, p. 677-680, October 2012.
A. Kamat, V. Oswal, and M. Datar, “Implementation of classification algorithms to predict mode of delivery,” International Journal of Computer Science and Information Technologies, vol. 6, no.5, pp. 4531-4534, 2015.
B. Lakshmi, T. Indumathi, and N. Ravi, “A comparative study of classification algorithms for predicting gestational risks in pregnant women,” International Conference on Computers, Communications, and Systems, November 2015, pp. 42-46.
R. Sawant and N. Gaikwad, “Hybrid prediction method for pregnancy data set,” 2015 1st International Conference on Next Generation Computing Technologies, September 2015, pp. 918-920.
R. Robu and Ş. Holban, “The analysis and classification of birth data,” Acta Polytechnica Hungarica, vol. 12, no. 4, pp. 77-96, July 2015.
S. A. Abbas, R. Riaz, S. Z. H. Kazmi, S. S. Rizvi, and S. J. Kwon, “Cause analysis of caesarian sections and application of machine learning methods for classification of birth data,” IEEE Access, November 2018, pp. 67555-67561.
M. R. Hassan, S. Al-Insaif, M. I. Hossain, and J. Kamruzzaman, “A machine learning approach for prediction of pregnancy outcome following IVF treatment,” Neural Computing and Applications, September 2018, pp. 1-15.
N. S. Prema and M. P. Pushpalatha, “Machine learning approach for preterm birth prediction based on maternal chronic conditions,” Emerging Research in Electronics, Computer Science and Technology, Springer, 2019.
N. S. Prema and M. P. Pushpalatha, “Prediction of preterm birth using data mining-a survey,” IIOAB Journal, vol. 10, no. 2, pp. 13-17, January 2019.
S. I. Ayon and M. Islam, “Diabetes prediction: A deep learning approach,” International Journal of Information Engineering and Electronic Business, vol. 2, pp. 21-27, March 2019.
M. M. Islam, H. Iqbal, M. R. Haque, and M. K. Hasan, “Prediction of breast cancer using support vector machine and K-nearest neighbors,” 2017 IEEE Region 10 Humanitarian Technology Conference (R10-HTC), December 2017, pp. 226-229.
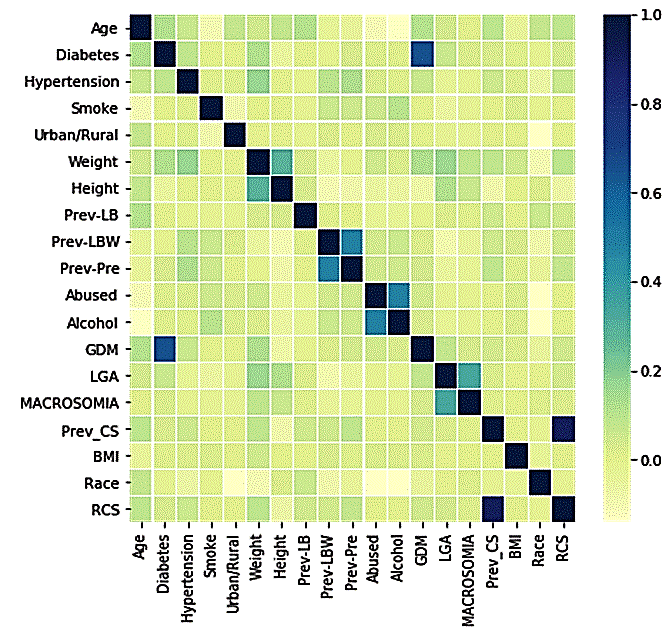
Published
How to Cite
Issue
Section
License
Submission of a manuscript implies: that the work described has not been published before that it is not under consideration for publication elsewhere; that if and when the manuscript is accepted for publication. Authors can retain copyright of their article with no restrictions. Also, author can post the final, peer-reviewed manuscript version (postprint) to any repository or website.
Since Oct. 01, 2015, PETI will publish new articles with Creative Commons Attribution Non-Commercial License, under The Creative Commons Attribution Non-Commercial 4.0 International (CC BY-NC 4.0) License.
The Creative Commons Attribution Non-Commercial (CC-BY-NC) License permits use, distribution and reproduction in any medium, provided the original work is properly cited and is not used for commercial purposes