Risk-Aware Multi-Agent Advantage Actor-Critic Based Resource Allocation for C-V2X Communication in Cellular Networks
DOI:
https://doi.org/10.46604/peti.2024.14136Keywords:
deep reinforcement learning, intelligent transportation systems, multi-agent, resource allocation, risk-awareAbstract
Intelligent transportation systems have emerged promisingly for industries to enable automated and safe driving. However, to satisfy reliability, environmental sustainability, and overall performance, deep reinforcement learning requires massive energy consumption with its computational demands. In this research, the risk-aware multi-agent advantage actor-critic (RA-MA-A2C)-based resource allocation (RA) is proposed for the cellular-vehicle-to-everything (C-V2X) network. The RA-MA-A2C considers collision risk when allocating resources such as frequency and power. By integrating risk assessment into the decision-making process, the RA-MA-A2C adjusts RA to mitigate collision risks and thereby increases the system’s effectiveness. The RA-MA-A2C’s performance is evaluated in terms of the success rate, completion time, vehicle-to-infrastructure link sum rate, and vehicle-to-vehicle links probability. The RA-MA-A2C demands 108 ms completion time with a 98.81% success rate, surpassing the performance of the existing offloading resource allocation-based deep reinforcement learning (ORAD) method.
References
H. Jin, J. Seo, J. Park, and S. C. Kim, “A Deep Reinforcement Learning-Based Two-Dimensional Resource Allocation Technique for V2I Communications,” IEEE Access, vol. 11, pp. 78867-78878, 2023.
T. I. Bayu, Y. F. Huang, and J. K. Chen, “Performance of Fuzzy Inference System for Adaptive Resource Allocation in C-V2X Networks,” Electronics, vol. 11, no. 23, article no. 4063, 2022.
S. M. Waqas, Y. Tang, L. Yu, and F. Abbas, “A Joint Cluster-Based RRM and Low-Latency Framework Using the Full-Duplex Mechanism for NR-V2X Networks,” Computer Communications, vol. 209, pp. 513-525, 2023.
S. Sabeeh and K. Wesołowski, “Congestion Control in Autonomous Resource Selection of Cellular-V2X,” IEEE Access, vol. 11, pp. 7450-7460, 2023.
N. Banitalebi, P. Azmi, N. Mokari, A. H. Arani, and H. Yanikomeroglu, “Distributed Learning-Based Resource Allocation for Self-Organizing C-V2X Communication in Cellular Networks,” IEEE Open Journal of the Communications Society, vol. 3, pp. 1719-1736, 2022.
Y. Ding, Y. Huang, L. Tang, X. Qin, and Z. Jia, “Resource Allocation in V2X Communications Based on Multi-Agent Reinforcement Learning with Attention Mechanism,” Mathematics, vol. 10, no. 19, article no. 3415, 2022.
Z. Li, K. Wang, T. Yu, and K. Sakaguchi, “Het-SDVN: SDN-Based Radio Resource Management of Heterogeneous V2X for Cooperative Perception,” IEEE Access, vol. 11, pp. 76255-76268, 2023.
G. Chai, W. Wu, Q. Yang, M. Qin, Y. Wu, and F. R. Yu, “Platoon Partition and Resource Allocation for Ultra-Reliable V2X Networks,” IEEE Transactions on Vehicular Technology, vol. 73, no. 1, pp. 147-161, 2024.
S. U. Nyati, S. R. Suralkar, and U. S. Bhadade, “V2X Spectrum Allocation for Emergency Communication Using Cognitive Radio Transmission,” SAMRIDDHI: A Journal of Physical Sciences, Engineering and Technology, vol. 14, no. 01, pp. 79-85, 2022.
D. Han and J. So, “Energy-Efficient Resource Allocation Based on Deep Q-Network in V2V Communications,” Sensors, vol. 23, no. 3, article no. 1295, 2023.
S. Feki, M. Hamdi, A. Belghith, F. Zarai, and A. D. Algarni, “Multi-Layer Neural Network Algorithm for Vehicle-to-Everything Communication in 5G Networks,” International Journal of Communication Systems, vol. 36, no. 7, article no. e5260, 2023.
R. H. Hwang, F. Marzuk, M. Sikora, P. Chołda, and Y. D. Lin, “Resource Management in LADNs Supporting 5G V2X Communications,” IEEE Access, vol. 11, pp. 63958-63971, 2023.
A. M. A. Ibrahim, Z. Chen, Y. Wang, H. A. Eljailany, and A. Ipaye, “Optimizing V2X Communication: Spectrum Resource Allocation and Power Control Strategies for Next-Generation Wireless Technologies,” Applied Sciences, vol. 14, no. 2, article no. 531, 2024.
P. Ma, P. Zhao, Z. Bai, X. Dong, X. Yang, and K. Kwak, “Coalitional Game Based Resource Allocation in D2D-Enabled V2V Communication,” Journal of Systems Engineering and Electronics, vol. 34, no. 6, pp. 1508-1519, 2023.
L. Shan, M. M. Wang, F. Zhang, S. Chen, and J. Zhang, “Resource Allocation for Cellular Device-to-Device-Aided Vehicle-to-Everything Networks with Partial Channel State Information,” Transactions on Emerging Telecommunications Technologies, vol. 33, no. 7, article no. e4501, 2022.
N. Zhao, J. Wang, B. Jin, R. Wang, M. Wu, Y. Liu, et al., “Multi-Agent Reinforcement Learning-Based Resource Management for V2X Communication,” International Journal of Mobile Computing and Multimedia Communications, vol. 14, no. 1, pp. 1-17, 2023.
Q. Liu, R. Luo, H. Liang, and Q. Liu, “Energy-Efficient Joint Computation Offloading and Resource Allocation Strategy for ISAC-Aided 6G V2X Networks,” IEEE Transactions on Green Communications and Networking, vol. 7, no. 1, pp. 413-423, 2023.
M. Christopoulou, S. Barmpounakis, H. Koumaras, and A. Kaloxylos, “Artificial Intelligence and Machine Learning as Key Enablers for V2X Communications: A Comprehensive Survey,” Vehicular Communications, vol. 39, article no. 100569, 2023.
M. Parvini, P. Schulz, and G. Fettweis, “Resource Allocation in V2X Networks: From Classical Optimization to Machine Learning-Based Solutions,” IEEE Open Journal of the Communications Society, vol. 5, pp. 1958-1974, 2024.
P. Hou, X. Jiang, Z. Lu, B. Li, and Z. Wang, “Joint Computation Offloading and Resource Allocation Based on Deep Reinforcement Learning in C-V2X Edge Computing,” Applied Intelligence, vol. 53, no. 19, pp. 22446-22466, 2023.
A. D. Mafuta, B. T. J. Maharaj, and A. S. Alfa, “Decentralized Resource Allocation-Based Multiagent Deep Learning in Vehicular Network,” IEEE Systems Journal, vol. 17, no. 1, pp. 87-98, 2023.
I. Lee and D. K. Kim, “Decentralized Multi-Agent DQN-Based Resource Allocation for Heterogeneous Traffic in V2X Communications,” IEEE Access, vol. 12, pp. 3070-3084, 2024.
S. M. Waqas, Y. Tang, F. Abbas, H. Chen, and M. Hussain, “A Novel Duplex Deep Reinforcement Learning Based RRM Framework for Next-Generation V2X Communication Networks,” Expert Systems with Applications, vol. 233, article no. 121004, 2023.
J. S. Alrubaye and B. S. Ghahfarokhi, “Geo-Based Resource Allocation for Joint Clustered V2I and V2V Communications in Cellular Networks,” IEEE Access, vol. 11, pp. 82601-82612, 2023.
N. Wan, Y. Luo, G. Zeng, and X. Zhou, “Minimization of VANET Execution Time Based on Joint Task Offloading and Resource Allocation,” Peer-to-Peer Networking and Applications, vol. 16, no. 1, pp. 71-86, 2023.
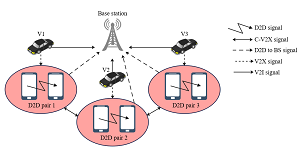
Published
How to Cite
Issue
Section
License
Copyright (c) 2025 Irshad Khan, Manjula Sunkadakatte Haladappa

This work is licensed under a Creative Commons Attribution-NonCommercial 4.0 International License.
Submission of a manuscript implies: that the work described has not been published before that it is not under consideration for publication elsewhere; that if and when the manuscript is accepted for publication. Authors can retain copyright of their article with no restrictions. Also, author can post the final, peer-reviewed manuscript version (postprint) to any repository or website.
Since Oct. 01, 2015, PETI will publish new articles with Creative Commons Attribution Non-Commercial License, under The Creative Commons Attribution Non-Commercial 4.0 International (CC BY-NC 4.0) License.
The Creative Commons Attribution Non-Commercial (CC-BY-NC) License permits use, distribution and reproduction in any medium, provided the original work is properly cited and is not used for commercial purposes