Personalized Rehabilitation Recognition Model upon ANFIS
DOI:
https://doi.org/10.46604/peti.2020.3912Keywords:
ANFIS, physiotherapy, rehabilitation recognition, sensor-enabled wristband, ubiquitous healthcareAbstract
This study applied the Adaptive Neuro-Fuzzy Inference System (ANFIS) to design a recognition model of personalized rehabilitation. In the model, the user may take a wearable sensor and follow the assigned joint-relax exercise to measure the motions of the upper limbs. The sensor that is embedded with the chips of accelerometer, gyroscope, and inclinometer produced the sample datasets due to the exercise schedule of physiotherapy assignment. All motion samples were labeled by arbitrary numbers, which can be identified to the specific motion, for the data training process. A Fuzzy Inference System (FIS) was initially designed by the steps of data pre-processing, featuring, fuzzifying, and ruling Fuzzy logics according to the sample datasets. The FIS was then trained by the ANFIS for optimization by tuning parameters of the features. In testing, the accomplished FIS could recognize the motion features by the defuzzifier that infers the label corresponding to the motion. As a result, the average recognition rate was higher than 90% when the testing motions followed the sampling schedule of the physiotherapy assignment. The model can be applied in the ubiquitous healthcare measurement for health services. The professionals can assess whether the subject obeyed the assigned program or not based on detail motions of the exercise. This approach can be enabled on the trackable interface for the physiatrists to screen the motions of routine rehabilitation.
References
M. Jackson, B. Michaud, P. Tétreault, and M. Begona, “Improvements in measuring shoulder joint kinematics,” Journal of Biomechanics, vol. 45, no. 12, pp. 2180-2183, June 2012.
Y. J. Chang, W. Y. Han, and Y. C. Tsai, “A Kinect-based upper limb rehabilitation system to assist people with cerebral palsy,” Research in Developmental Disabilities, vol. 34, no. 11, pp. 3654-3659, September 2013.
Darwish and A. E. Hassanien, “Wearable and implantable wireless sensor network solutions for healthcare monitoring,” Sensors, vol. 11, no. 6, pp. 5561-5595, May 2011.
M. Chen, S. Gonzalez, A. Vasilakos, H. Cao, and V. Leung, “Body area networks: a survey,” Mobile Networks and Applications (MONET). Springer Netherlands. vol. 16, no. 2, pp. 171-193, April 2011.
M. Singh and S. K. Soni, “Spatial correlation-based clustering in wireless sensor network,” International Journal of Engineering and Technology Innovation, vol. 8, no. 4, pp. 294-306, January 2018.
P. Mandal, K. Tank, T. Mondal, C. H. Chen, and M. J. Deen, “Predictive walking-age health analyzer,” IEEE Journal Biomedical and Health Informatics, vol. 22, no. 2, pp. 363-374, March 2018.
R. R. Fletcher, M. Z. Poh, and H. Eydgahi, “Wearable sensors: opportunities and challenges for low-cost health care,” in 2010 Annual International Conference of the IEEE Engineering in Medicine and Biology, Buenos Aires, Argentina, September 2010, pp. 1763-1766.
K. Nguyen-Huu, C. G. Song, and S. W. Lee, “Smartwatch/smartphone cooperative indoor lifelogging system,” International Journal of Engineering and Technology Innovation, vol. 8, no. 4, pp. 261-273, September 2018.
C. C. Lee, “Fuzzy logic in control systems: fuzzy logic controller: part II,” IEEE Trans. on Systems, Man, and Cybernetics, vol. 20, no. 2, pp. 419-435, 1990.
L. A. Zadeh, “Outline of a new approach to the analysis of complex systems and decision processes,” IEEE Transactions on Systems, Man, and Cybernetics, vol. 3, no. 1, pp. 28-44, January 1973.
L. Li, Y. Ma, B. Wang, H. Dong, and Z. Zhang, “Research on traffic signal timing method based on ant colony algorithm and fuzzy control theory,” in Proceedings of Engineering and Technology Innovation, vol. 11, pp. 21-29, 2019.
E. H. Mamdani and S. Assilian, “An experiment in linguistic synthesis with a fuzzy logic controller,” International Journal of Man-Machine Studies, vol. 7, no. 1, pp. 1-13, January 1975.
T. Takagi and M. Sugeno, “Derivation of fuzzy control rules from human operator’s control actions,” Proc. of the IFAC Symp. on Fuzzy Information, Knowledge Representation, and Decision Analysis, Marseilles, France, July 1983, pp. 55-60.
J. S. R. Jang, “ANFIS: adaptive-network-based fuzzy inference system,” IEEE Transaction on Systems, Man, and Cybernetics, vol. 23, no. 3, pp. 665-685, 1993.
F. Attal, S. Mohammed, M. Dedabrishvili, F. Chamroukhi, L. Oukhellou, and Y. Amirat, “Physical human activity recognition using wearable sensors,” Sensors (Basel), vol. 15, no. 12, pp. 31314-31338, December 2015. DOI: 10.3390/s151229858
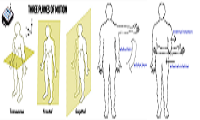
Published
How to Cite
Issue
Section
License
Submission of a manuscript implies: that the work described has not been published before that it is not under consideration for publication elsewhere; that if and when the manuscript is accepted for publication. Authors can retain copyright of their article with no restrictions. Also, author can post the final, peer-reviewed manuscript version (postprint) to any repository or website.
Since Oct. 01, 2015, PETI will publish new articles with Creative Commons Attribution Non-Commercial License, under The Creative Commons Attribution Non-Commercial 4.0 International (CC BY-NC 4.0) License.
The Creative Commons Attribution Non-Commercial (CC-BY-NC) License permits use, distribution and reproduction in any medium, provided the original work is properly cited and is not used for commercial purposes