Mixed Noise Removal by Processing of Patches
DOI:
https://doi.org/10.46604/peti.2020.6082Keywords:
speckle, patches, blind denoising, blocksAbstract
Sonar images are degraded by mixed noise which has an adverse impact on detection and classification of underwater objects. Existing denoising methods of sonar images remove either additive noise or multiplicative noise. In this study, the mixed noise in sonar images, the additive Gaussian noise and the multiplicative speckle effect are handled by the data adaptive methods. A patch based denoising is applied in two phases to remove the additive Gaussian and multiplicative speckle noises. In the first phase, the adaptive processing of local patches is used to remove the additive Gaussian noise by exploiting the sonar image local sparsity. The PCA and SVD methods are used for denoising the noisy image patches and blocks of patches. In the second phase, the weighted maximum likelihood denoising of the nonlocal patches reduces the speckle effect by exploiting the non-local similarity in a probability distribution. Experiments on side scan sonar images are conducted and the results show the importance of removing both the additive and multiplicative components from the sonar images.
References
P. Blondel, The Handbook of Sidescan Sonar, 1st ed. UK: Springer Praxis Publishing, 2009.
J. W. Goodman, “Some Fundamental Properties of Speckle,” Journal of Optical Society of America, vol. 66, no. 11, pp. 1145-1150, 1976.
A. K. Jain, Fundamentals of Digital Processing, USA: Prentice-Hall, Inc, 1989.
F. Maussang, J. Chanussot, A. Htet, and M. Amate, “Mean-Standard Deviation Representation of Sonar Images for Echo Detection: Application to SAS Images,” IEEE Journal of Oceanic Engineering, vol. 32, no. 4, October 2007.
A. Achim, A. Bezerianos, and P. Tsakalides, “Novel Bayesian Multiscale Method for Speckle Removal in Medical Ultrasound Images,” IEEE Transacton Medical Imaging, vol. 20, no. 8, pp. 772-783, August 2001.
R. W. Liu, Y. Lu, F. Chen, and L. Xie, “Learning a Deep Convolutional Network for Speckle Noise Reduction in Underwater Sonar Images,” Proceedings of the 2019 11th International Conference on Machine Learning and Computing, February 2019, pp. 445-450.
U. Anithaa, S. Malarkkanb, G. D. Anbarasi Jebaselvia, and R. Narmadhaa, “Sonar Image Segmentation and Quality Assessment Using Prominent Image Processing Techniques,” Applied Acoustics, vol. 148, pp. 300-307, May 2019.
G. Li, F. Zhang, and Q. Liu, “Distribution-Transformed Network for Impulse Noise Removal,” Journal of Shanghai Jiaotong University (Science), June 2020.
N. H. T. Dang, S. D. Dvoenko, and V. S. Dinh, “A Mixed Noise Removal Method Based on Total Variation,” Informatica, vol. 40, no. 2, pp. 159-167, July 2016.
T. Yamaguchi, A. Suzuki, and M. Ikehara, “Detail Preserving Mixed Noise Removal by DWM Filter and BM3D,” IEICE Transactions on Fundamentals of Electronics, Communications and Computer Sciences, vol. EI00-A, no. 11, pp. 2451-2457, November 2017.
X. Liu, M. Tanaka, and M. Okutomi, “Single-Image Noise Level Estimation for Blind Denoising,” IEEE Transactions on Image Processing,” vol. 22, no. 12, pp. 5226-5237, December 2013.
S. Pyatykh, J. Hesser, and L. Zheng “Image Noise Level Estimation by Principal Component Analysis,” IEEE Transactions on Image Processing, vol. 22, no. 2, pp. 687-699, February 2013.
C. M. Bishop, Pattern Recognition and Machine Learning, New York: Springer, 2006.
V. P. S. Naidu and J. R. Raol, “Pixel-level Image Fusion Using Wavelets and Principal Component Analysis,” Defense Science Journal, vol. 58, no. 3, pp. 338-352, March 2008.
R. James and M.H. Supriya, “Sonar Image Denoising using Adaptive Processing of Local Patches,” 2015 International Symposium on Ocean Electronics (SYMPOL), IEEE Press, November 2015, pp. 1-7.
Y. He, T. Gan, W. Chen, and H. Wang, “Adaptive Denoising by Singular Value Decomposition,” IEEE Signal Processing Letters, vol. 18, no. 4, pp. 215-218, April 2011.
C. A. Deledalle, L. Denis, and F. Tupin, “Iterative Weighted Maximum Likelihood Denoising with Probabilistic Patch-Based Weights,” IEEE Transactions on Image Processing, vol. 18, no. 12, pp. 2661-2672, December 2009.
A. Buades, B. Coll, and J. Morel “A Non-Local Algorithm for Image Denoising,” 2005 IEEE Computer Society Conference on Computer Vision and Pattern Recognition (CVPR'05), IEEE Press, July 2005, pp. 60-65.
C. Kervrann, P. Perez, and J. Boulanger, “Bayesian Non-local Means Image Redundancy and Adaptive Estimation for Image Representation and Applications,” SIAM Conference on Imaging Science, July 2008.
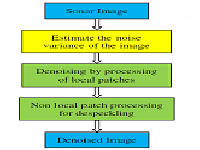
Published
How to Cite
Issue
Section
License
Submission of a manuscript implies: that the work described has not been published before that it is not under consideration for publication elsewhere; that if and when the manuscript is accepted for publication. Authors can retain copyright of their article with no restrictions. Also, author can post the final, peer-reviewed manuscript version (postprint) to any repository or website.
Since Oct. 01, 2015, PETI will publish new articles with Creative Commons Attribution Non-Commercial License, under The Creative Commons Attribution Non-Commercial 4.0 International (CC BY-NC 4.0) License.
The Creative Commons Attribution Non-Commercial (CC-BY-NC) License permits use, distribution and reproduction in any medium, provided the original work is properly cited and is not used for commercial purposes