A CNN Based Approach for Garments Texture Design Classification
Keywords:
CNN, deep learning, AlexNet, VGGNet, texture de-scriptor, garment categories, garment trend identification, design classification for garmentsAbstract
Identifying garments texture design automatically for recommending the fashion trends is important nowadays because of the rapid growth of online shopping. By learning the properties of images efficiently, a machine can give better accuracy of classification. Several Hand-Engineered feature coding exists for identifying garments design classes. Recently, Deep Convolutional Neural Networks (CNNs) have shown better performances for different object recognition. Deep CNN uses multiple levels of representation and abstraction that helps a machine to understand the types of data more accurately. In this paper, a CNN model for identifying garments design classes has been proposed. Experimental results on two different datasets show better results than existing two well-known CNN models (AlexNet and VGGNet) and some state-of-the-art Hand-Engineered feature extraction methods.
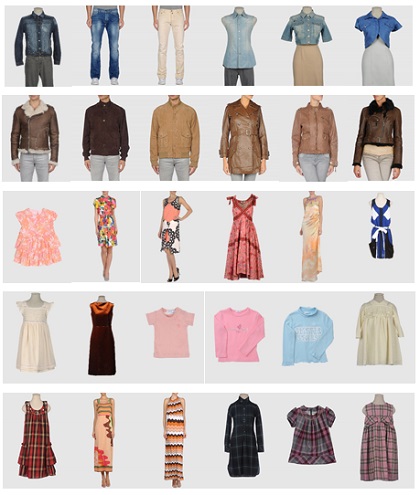
Published
How to Cite
Issue
Section
License
Submission of a manuscript implies: that the work described has not been published before that it is not under consideration for publication elsewhere; that if and when the manuscript is accepted for publication. Authors can retain copyright in their articles with no restrictions. is accepted for publication. Authors can retain copyright of their article with no restrictions.
Since Jan. 01, 2019, AITI will publish new articles with Creative Commons Attribution Non-Commercial License, under The Creative Commons Attribution Non-Commercial 4.0 International (CC BY-NC 4.0) License.
The Creative Commons Attribution Non-Commercial (CC-BY-NC) License permits use, distribution and reproduction in any medium, provided the original work is properly cited and is not used for commercial purposes.