Optimizing Lags and Hidden Layers in Hybrid Models for Forecasting Stock Return
DOI:
https://doi.org/10.46604/aiti.2024.14192Keywords:
econometrics, hybrid model, ANN, ARIMAX, ERNNAbstract
This study aims to minimize the root mean square error for stock return by optimizing lags and hidden layers in a hybrid model. The model combines the autoregressive integrated moving average with the exogenous variables model as linear components. The residuals derived from linear components are used in artificial neural networks and Elman recurrent neural networks as non-linear components. A key feature of this approach is optimizing the selection of hidden layers and lags within the neural network, improving forecasting accuracy. The minimum mean square error forecast expression is derived, and the model is tested on stock price data during the COVID-19 period, marked by significant market shocks. The root mean square error results for the proposed model, traditional hybrid model, and traditional time series model range from 0.0004 to 0.01, 0.0006 to 0.01, and 0.006 to 0.03, respectively. The results show that the proposed model outperforms both traditional models.
References
K. A. Ababio, “Comparative Study of Stock Price Forecasting Using ARIMA and ARIMAX Models,” Ph.D. dissertation, Department of Mathematics, Kwame Nkrumah University of Science and Technology, Kumasi, 2012.
D. K. Shetty and B. Ismail, “Forecasting Stock Prices Using Hybrid Non-Stationary Time Series Model With ERNN,” Communications in Statistics-Simulation and Computation, vol. 52, no. 3, pp. 1026-1040, 2023.
P. Almaleck, S. Massucco, G. Mosaico, M. Saviozzi, P. Serra, and F. Silvestro, “Electrical Consumption Forecasting in Sports Venues: A Proposed Approach Based on Neural Networks and ARIMAX Models,” Sustainable Cities and Society, vol. 100, article no. 105019, 2024.
A. A. Pierre, S. A. Akim, A. K. Semenyo, and B. Babiga, “Peak Electrical Energy Consumption Prediction by ARIMA, LSTM, GRU, ARIMA-LSTM and ARIMA-GRU Approaches,” Energies, vol. 16, no. 12, article no. 4739, 2023.
D. Xu, Q. Zhang, Y. Ding, and D. Zhang, “Application of a Hybrid ARIMA-LSTM Model Based on the SPEI for Drought Forecasting,” Environmental Science and Pollution Research, vol. 29, no. 3, pp. 4128-4144, 2022.
A. S. Azad, R. Sokkalingam, H. Daud, S. K. Adhikary, H. Khurshid, S. N. A. Mazlan, et al., “Water Level Prediction through Hybrid SARIMA and ANN Models Based on Time Series Analysis: Red Hills Reservoir Case Study,” Sustainability, vol. 14, no. 3, article no. 1843, 2022.
K. Nualtong, T. Panityakul, P. Khwanmuang, R. Chinram, and S. Kirtsaeng, “A Hybrid Seasonal Box Jenkins-ANN Approach for Water Level Forecasting in Thailand,” Environment and Ecology Research, vol. 9, no. 3, pp. 93-106, 2021.
S. A. Shahriar, I. Kayes, K. Hasan, M. Hasan, R. Islam, N. R. Awang, et al., “Potential of ARIMA-ANN, ARIMA-SVM, DT and CatBoost for Atmospheric PM2.5 Forecasting in Bangladesh,” Atmosphere, vol. 12, no. 1, article no.. 100, 2021.
D. Fan, H. Sun, J. Yao, K. Zhang, X. Yan, and Z. Sun, “Well Production Forecasting Based on ARIMA-LSTM Model Considering Manual Operations,” Energy, vol. 220, article no. 119708, 2021.
M. Wang, J. Pan, X. Li, M. Li, Z. Liu, Q. Zhao, et al., “ARIMA and ARIMA-ERNN Models for Prediction of Pertussis Incidence in Mainland China From 2004 to 2021,” BMC Public Health, vol. 22, no. 1, article no. 1447, 2022.
A. Sharma, P. Tiwari, A. Gupta, and P. Garg, “Use of LSTM and ARIMAX Algorithms to Analyze Impact of Sentiment Analysis in Stock Market Prediction,” Intelligent Data Communication Technologies and Internet of Things: Proceedings of ICICI 2020, pp. 377-394, 2021.
P. Lv, Q. Wu, J. Xu, and Y. Shu, “Stock Index Prediction Based on Time Series Decomposition and Hybrid Model,” Entropy, vol. 24, no. 2, article no. 146, 2022.
E. Alshawarbeh, A. T. Abdulrahman, and E. Hussam, “Statistical Modeling of High Frequency Datasets Using the ARIMA-ANN Hybrid,” Mathematics, vol. 11, no. 22, article no. 4594, 2023.
B. Singh, S. K. Henge, S. K. Mandal, M. K. Yadav, P. T. Yadav, A. Upadhyay, et al., “Auto-Regressive Integrated Moving Average Threshold Influence Techniques for Stock Data Analysis,” International Journal of Advanced Computer Science and Applications, vol. 14, no. 6, pp. 446-455, 2023.
“SET Industry Group and Sector Classification,” https://www.set.or.th/en/market/index/set/industry-sector-profile, accessed on 2021.
N. Hongsakulvasu, C. Khiewngamdee, and A. Liammukda, “Does COVID-19 Crisis Affects the Spillover of Oil Market’s Return and Risk on Thailand’s Sectoral Stock Return?: Evidence from Bivariate DCC GARCH-in-Mean Model,” International Energy Journal, vol. 20, no. 4, pp. 647-662, 2020.
P. C. B. Phillips and P. Perron, “Testing for a Unit Root in Time Series Regression,” Biometrika, vol. 75, no. 2, pp. 335-346, 1988.
D. F. Chang, C. C. Chen, and A. Chang, “Forecasting With ARIMAX Models for Participating STEM Programs,” ICIC Express Letters. Part B, Applications, vol. 11, no. 2, pp. 121-128, 2020.
C. H. Aladag, E. Egrioglu, and C. Kadilar, “Forecasting Nonlinear Time Series With a Hybrid Methodology,” Applied Mathematics Letters, vol. 22, no. 9, pp. 1467-1470, 2009.
J. D. Cryer and K. S. Chan, Time Series Analysis: With Applications in R, 2nd ed., New York: Springer, 2008.
T. Guillod, P. Papamanolis, and J. W. Kolar, “Artificial Neural Network (ANN) Based Fast and Accurate Inductor Modeling and Design,” IEEE Open Journal of Power Electronics, vol. 1, pp. 284-299, 2020.
S. Stephen, O. Argwings, and K. Julius, “Application of ARIMA, Hybrid ARIMA and Artificial Neural Network Models in Predicting and Forecasting Tuberculosis Incidences Among Children in Homa Bay and Turkana Counties, Kenya,” in press. https://doi.org/10.1101/2022.07.07.22277378
J. Wang, J. Wang, W. Fang, and H. Niu, “Financial Time Series Prediction Using Elman Recurrent Random Neural Networks,” Computational Intelligence and Neuroscience, vol. 2016, no. 1, article no. 4742515, 2016.
D. Zhang, W. Li, X. Han, B. Lu, Q. Zhang, and C. Bo, “Evolving Elman Neural Networks Based State-of-Health Estimation for Satellite Lithium-Ion Batteries,” Journal of Energy Storage, vol. 59, article no. 106571, 2023.
K. Sako, B. N. Mpinda, and P. C. Rodrigues, “Neural Networks for Financial Time Series Forecasting,” Entropy, vol. 24, no. 5, article no. 657, 2022.
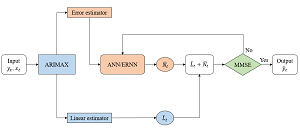
Published
How to Cite
Issue
Section
License
Copyright (c) 2025 Nuttaphat Sukchitt, Manad Khamkong, Lampang Saechan, Napon Hongsakulvasu

This work is licensed under a Creative Commons Attribution-NonCommercial 4.0 International License.
Submission of a manuscript implies: that the work described has not been published before that it is not under consideration for publication elsewhere; that if and when the manuscript is accepted for publication. Authors can retain copyright in their articles with no restrictions. is accepted for publication. Authors can retain copyright of their article with no restrictions.
Since Jan. 01, 2019, AITI will publish new articles with Creative Commons Attribution Non-Commercial License, under The Creative Commons Attribution Non-Commercial 4.0 International (CC BY-NC 4.0) License.
The Creative Commons Attribution Non-Commercial (CC-BY-NC) License permits use, distribution and reproduction in any medium, provided the original work is properly cited and is not used for commercial purposes.