Biosignal –Based Multimodal Biometric System
Keywords:
biometric, pattern recognition, per-sonal identificationAbstract
This study concerns personal identification based on electrocardiogram (ECG) and photoplethysmogram (PPG) signals. We manufactured a bio-signal measurement system that can simultaneously measure ECG and PPG signals, using which three channels of ECG signal and one channel of PPG signal were acquired from the right-hand index finger of a total of 33 subsets for 3 minutes. Lead-I signal of the three-channel ECG signal and the one-channel PPG signal were selected for recognition. For each subject, 160 heartbeats were automatically separated from the acquired bio-signals, and a total of 21 features, comprising 15 ECG features, 4 ECG-related PPG features, and 2 features concerning PPG only, were extracted from each heartbeat. Letting the 21 features form a single data point, heartbeat features of each subset were used as the training data for a support vector machine (SVM) classifier, with the number of data points being adjusted from 10 to 80, and the data points (80 - 150) other than the training data were used as the testing data, in order to investigate the recognition performance indices. As a result, the proposed algorithm showed high recognition performance of 99.28% accuracy, 0.88% FRR, 0.85% FAR, 99.28% sensitivity, and 99.31% specificity, when there are 80 training data points. Moreover, even when there are 10 training data points, the proposed algorithm showed the performance of 92.77% accuracy, 7.23% FRR, 6.29% FAR, 92.77% sensitivity, and 93.21% specificity, which can be evaluated as an extremely high recognition performance considering that there was a total of 4,950 testing data points.
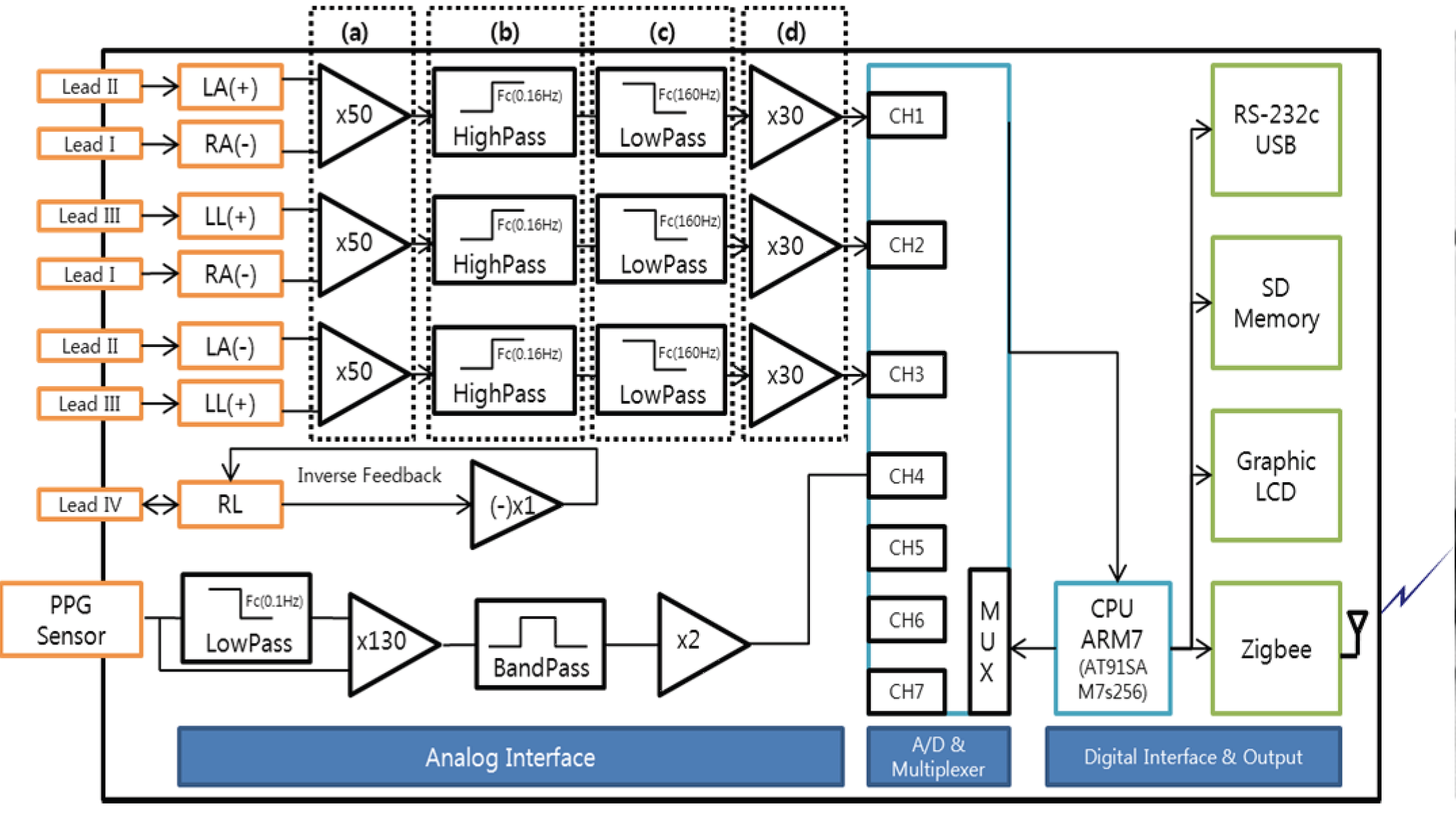
Published
How to Cite
Issue
Section
License
Submission of a manuscript implies: that the work described has not been published before that it is not under consideration for publication elsewhere; that if and when the manuscript is accepted for publication. Authors can retain copyright in their articles with no restrictions. is accepted for publication. Authors can retain copyright of their article with no restrictions.
Since Jan. 01, 2019, AITI will publish new articles with Creative Commons Attribution Non-Commercial License, under The Creative Commons Attribution Non-Commercial 4.0 International (CC BY-NC 4.0) License.
The Creative Commons Attribution Non-Commercial (CC-BY-NC) License permits use, distribution and reproduction in any medium, provided the original work is properly cited and is not used for commercial purposes.