A Hidden Semi-Markov Model for Predicting Production Cycle Time Using Bluetooth Low Energy Data
DOI:
https://doi.org/10.46604/aiti.2023.11678Keywords:
Bluetooth low energy, received signal strength indicator, hidden semi-Markov model, learning problemAbstract
This study proposes a statistical model to characterize the temporal characteristics of an entire production process. The model utilizes received signal strength indicator (RSSI) data obtained from a Bluetooth low energy (BLE) network. A hidden semi-Markov model (HSMM) is formulated based on the characteristics of the production process, and the forward-backward algorithm is employed to re-estimate the probability distribution of state durations. The proposed method is validated through numerical, simulation, and real-world experiments, yielding promising results. The results show that the Kullback-Leibler divergence (KLD) score of 0.1843, while the simulation achieves an average vector distance score of 0.9740. The real-time experiment also shows a reasonable accuracy, with an average HSMM estimated throughput time of 30.48 epochs, compared to the average real throughput time of 33.99 epochs. Overall, the model serves as a valuable tool for predicting the cycle time and throughput time of a production line.
References
W. C. Tan and M. S. Sidhu, “Review of RFID and IoT Integration in Supply Chain Management,” Operations Research Perspectives, vol. 9, article no. 100229, January 2022.
W. C. Chen, M. H. Nguyen, and P. H. Tai, “An Intelligent Manufacturing System for Injection Molding,” Proceedings of Engineering and Technology Innovation, vol. 8, pp. 09-14, April 2018.
J. V. Tébar-Rubio, F. J. Ramírez, and M. J. Ruiz-Ortega, “Conducting Action Research to Improve Operational Efficiency in Manufacturing: The Case of a First-Tier Automotive Supplier,” Systemic Practice and Action Research, vol. 36, no. 3, pp. 427-459, June 2023.
R. Joppen, S. Von Enzberg, J. Gundlach, A. Kühn, and R. Dumitrescu, “Key Performance Indicators in the Production of the Future,” Procedia CIRP, vol. 81, pp. 759-764, 2019.
S. Gao, J. I. U. Rubrico, T. Higashi, T. Kobayashi, K. Taneda, and J. Ota, “Efficient Throughput Analysis of Production Lines Based on Modular Queues,” IEEE Access, vol. 7, pp. 95314-95326, July 2019.
P. Bencak, D. Hercog, and T. Lerher, “Indoor Positioning System Based on Bluetooth Low Energy Technology and a Nature-Inspired Optimization Algorithm,” Electronics, vol. 11, no. 3, article no. 308, February 2022.
S. Vorapojpisut and K. Agrawal, “Modeling of Manufacturing Processes Using Hidden Semi-Markov Model and RSSI Data,” 17th International Joint Symposium on Artificial Intelligence and Natural Language Processing, November 2022.
T. Kluge, C. Groba, and T. Springer, “Trilateration, Fingerprinting, and Centroid: Taking Indoor Positioning with Bluetooth LE to the Wild,” 21st International Symposium on “A World of Wireless, Mobile and Multimedia Networks”, August-September 2020.
D. Han, H. Rho, and S. Lim, “HMM-Based Indoor Localization Using Smart Watches’ BLE Signals,” 6th International Conference on Future Internet of Things and Cloud, pp. 296-302, August 2018.
M. Arslan, C. Cruz, and D. Ginhac, “Semantic Trajectory Insights for Worker Safety in Dynamic Environments,” Automation in Construction, vol. 106, article no. 102854, October 2019.
S. Sun, Y. Li, W. S. T. Rowe, X. Wang, A. Kealy, and B. Moran, “Practical Evaluation of a Crowdsourcing Indoor Localization System Using Hidden Markov Models,” IEEE Sensors Journal, vol. 19, no. 20, pp. 9332-9340, October 2019.
S. Sun, X. Wang, B. Moran, A. Al-Hourani, and W. S. T. Rowe, “Radio Source Localization Using Received Signal Strength in a Multipath Environment,” 22nd International Conference on Information Fusion, pp. 1-6, July 2019.
S. Sun, X. Wang, B. Moran, and W. S. T. Rowe, “A Hidden Semi-Markov Model for Indoor Radio Source Localization Using Received Signal Strength,” Signal Processing, vol. 166, article no. 107230, January 2020.
C. H. Lin, K. J. Wang, A. A. Tadesse, and B. H. Woldegiorgis, “Human-Robot Collaboration Empowered by Hidden Semi-Markov Model for Operator Behaviour Prediction in a Smart Assembly System,” Journal of Manufacturing Systems, vol. 62, pp. 317-333, January 2022.
W. Yang and L. Chen, “Machine Condition Recognition via Hidden Semi-Markov Model,” Computers & Industrial Engineering, vol. 158, article no. 107430, August 2021.
B. Mor, S. Garhwal, and A. Kumar, “A Systematic Review of Hidden Markov Models and Their Applications,” Archives of Computational Methods in Engineering, vol. 28, no. 3, pp. 1429-1448, May 2021.
K. Li, C. Qiu, X. Zhou, M. Chen, Y. Lin, X. Jia, et al., “Modeling and Tagging of Time Sequence Signals in the Milling Process Based on an Improved Hidden Semi-Markov Model,” Expert Systems with Applications, vol. 205, article no. 117758, November 2022.
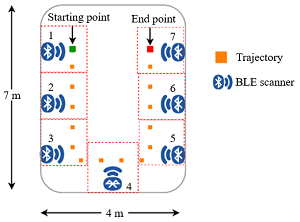
Published
How to Cite
Issue
Section
License
Copyright (c) 2023 Karishma Agrawal, Supachai Vorapojpisut

This work is licensed under a Creative Commons Attribution-NonCommercial 4.0 International License.
Submission of a manuscript implies: that the work described has not been published before that it is not under consideration for publication elsewhere; that if and when the manuscript is accepted for publication. Authors can retain copyright in their articles with no restrictions. is accepted for publication. Authors can retain copyright of their article with no restrictions.
Since Jan. 01, 2019, AITI will publish new articles with Creative Commons Attribution Non-Commercial License, under The Creative Commons Attribution Non-Commercial 4.0 International (CC BY-NC 4.0) License.
The Creative Commons Attribution Non-Commercial (CC-BY-NC) License permits use, distribution and reproduction in any medium, provided the original work is properly cited and is not used for commercial purposes.