Developing and Implementing an AI-Based Leak Detection System in a Long-Distance Gas Pipeline
DOI:
https://doi.org/10.46604/aiti.2022.8904Keywords:
artificial intelligence, convolutional neural network, pipeline leak, leak detectionAbstract
This research proposes an artificial intelligence (AI) detection model using convolutional neural networks (CNN) to automatically detect gas leaks in a long-distance pipeline. The change of gap pressure is collected when leakage occurs in the pipeline, and thereby the feature of gas leakage is extracted for building the CNN model. The gas leak patterns in the long-distance pipeline are analyzed. A pipeline detection model based on AI technology for automatically monitoring the leaks is proposed by extracting the feature of gas leakage. This model is tested by collecting gas pressure data from an existing natural gas pipeline system starting from Mailiao to Taoyuan in Taiwan. The testing result shows that the reduced model of leak detection can be used to detect the leaks from the upstream and downstream pipelines, and the AI-based pipeline leak detection system can obtain a satisfactory result.
References
M. Meribout, et al., “Leak Detection Systems in Oil and Gas Fields: Present Trends and Future Prospects,” Flow Measurement and Instrumentation, vol. 75, Article no. 101772, October 2020.
L. Yang, et al., “A Novel PPA Method for Fluid Pipeline Leak Detection Based on OPELM and Bidirectional LSTM,” IEEE Access, vol. 8, pp. 107185-107199, 2020.
M. Zhou, et al., “Leak Detection and Location Based on ISLMD and CNN in a Pipeline,” IEEE Access, vol. 7, pp. 30457-30464, 2019.
R. O. Melo, et al., “Applying Convolutional Neural Networks to Detect Natural Gas Leaks in Wellhead Images,” IEEE Access, vol. 8, pp. 191775-191784, 2020.
J. Li, et al., “A Small Leakage Detection Approach for Gas Pipelines Based on CNN,” 2019 CAA Symposium on Fault Detection, Supervision, and Safety for Technical Processes, pp. 390-394, July 2019.
T. Kattenborn, et al., “Review on Convolutional Neural Networks (CNN) in Vegetation Remote Sensing,” ISPRS Journal of Photogrammetry and Remote Sensing, vol. 173, pp. 24-49, March 2021.
J. Kang, et al., “Novel Leakage Detection by Ensemble CNN-SVM and Graph-Based Localization in Water Distribution Systems,” IEEE Transactions on Industrial Electronics, vol. 65, no. 5, pp. 4279-4289, May 2018.
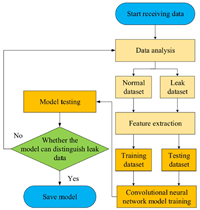
Published
How to Cite
Issue
Section
License
Submission of a manuscript implies: that the work described has not been published before that it is not under consideration for publication elsewhere; that if and when the manuscript is accepted for publication. Authors can retain copyright in their articles with no restrictions. is accepted for publication. Authors can retain copyright of their article with no restrictions.
Since Jan. 01, 2019, AITI will publish new articles with Creative Commons Attribution Non-Commercial License, under The Creative Commons Attribution Non-Commercial 4.0 International (CC BY-NC 4.0) License.
The Creative Commons Attribution Non-Commercial (CC-BY-NC) License permits use, distribution and reproduction in any medium, provided the original work is properly cited and is not used for commercial purposes.