Analysis of Feature Extraction Methods for Speaker Dependent Speech Recognition
Keywords:
Speech recognition, Feature Extraction, Mel-Frequency Cepstral Coefficients, Linear Predictive Coding Coefficients, Perceptual Linear Production, RASTA-PLP, Isolated words.Abstract
Speech recognition is about what is being said, irrespective of who is saying. Speech recognition is a growing field. Major progress is taking place on the technology of automatic speech recognition (ASR). Still, there are lots of barriers in this field in terms of recognition rate, background noise, speaker variability, speaking rate, accent etc. Speech recognition rate mainly depends on the selection of features and feature extraction methods. This paper outlines the feature extraction techniques for speaker dependent speech recognition for isolated words. A brief survey of different feature extraction techniques like Mel-Frequency Cepstral Coefficients (MFCC), Linear Predictive Coding Coefficients (LPCC), Perceptual Linear Prediction (PLP), Relative Spectra Perceptual linear Predictive (RASTA-PLP) analysis are presented and evaluation is done. Speech recognition has various applications from daily use to commercial use. We have made a speaker dependent system and this system can be useful in many areas like controlling a patient vehicle using simple commands.
References
D. Shaughnessy, “Invited paper: automatic speech recognition: history, methods and challenges,” Pattern Recognition, vol. 41, no. 10, pp. 2965-2979, 2008.
M. Benzeghiba, R. De Mori, O. Deroo, S. Dupont, T. Erbes, D. Jouvet, et. al, “Automatic speech recognition and speech variability: a review,” Speech Communication, vol. 49, no. 10-11, pp. 763-786, 2007.
N. Singh, R. A. Khan and R. Shree, “Applications of speaker recognition,” Procedia Engineering, vol. 38, pp. 3122-3126, 2012.
S. Furui, “50 years of progress in speech and speaker recognition,” ECTI Transactions on Computer and Information Technology, vol. 1, no. 2, 2005.
N. Alee, P. Ehkan, R. Badlishah Ahmad, and N. Sabri, “Speaker recognition system: vulnerable and challenges,” International Journal of Engineering and Technology, vol. 5, no. 4, pp. 3191-3195, 2013.
M. E. Ayadi, M. S. Kamel, F. Karray, “Survey on speech emotion recognition: Features, classification schemes, and databases,” Pattern Recognition, vol. 44, no. 3, pp. 572-587, 2011.
V. Fontaine and H. Bourlard, “Speaker-dependent speech recognition based on phone-like units models-application to voice dialing,” Proceeding IEEE International Conference on Acoustic Speech, Signal Processing, vol. 2, pp. 1527-1530, 1997.
X. Huang and K. F. Lee, “On speaker-independent, speaker-dependent, and speaker-adaptive speech recognition,” IEEE Transaction on Speech audio Processing, vol. 1, no. 2, pp. 150-157, 1993.
P. Cerva, J. Silovsky, J. Zdansky, J. Nouza, and L. Seps, “Speaker-adaptive speech recognition using speaker diarization for improved transcription of large spoken archives,” Speech Communication, vol. 55, no. 10, pp. 1033-1046, 2013.
L. Wang, J. Wang, L. Li, T. F. Zheng, and F. K. Soong, “Improving speaker verification performance against long-term speaker variability,” Speech Communication, vol. 79, no. C, pp. 14-29, 2016.
O. Scharenborg, “Reaching over the gap: a review of efforts to link human and automatic speech recognition research,” Speech Communication, vol. 49, no. 5, pp. 336-347, 2007.
N. S. Dey, R. Mohanty, and K. L. Chugh, “Speech and speaker recognition system using artificial neural networks and hidden markov model,” International Conference on Communication System and Network Technology, pp. 311-315, 2012.
R. Makhijani, and R. Gupta, “Isolated word speech recognition system using dynamic time warping,” International Journal of Engineering sciences and emerging Technologies, vol. 6, no. 3, pp. 352-367, 2013.
F. Guojiang, “A novel isolated speech recognition method based on neural network,” International Conference on Networking and Information Technology, vol. 17, pp. 264-269, 2011.
H. Ali, N. Ahmad, X. Zhou, K. Iqbal, and S. M. Ali, “DWT features performance analysis for automatic speech recognition of Urdu,” Springerplus, vol. 3, pp. 1-10, 2014.
B. C. Kamble, “Speech recognition using artificial neural network,” International Journal of Computing, Communications & Instrumentation Engineering, vol. 3, pp. 1-4, 2016.
R. Bharti, “Real time speaker recognition system using MFCC and vector quantization technique,” International Journal of Computer Applications, vol. 117, no. 1, pp. 25-31, 2015.
V. Z. Kepuska and H. A. Elharati, “Robust speech recognition system using conventional and hybrid features of MFCC, LPCC, PLP, RASTA-PLP and hidden markov model classifier in noisy conditions,” Journal of Computer and Communications, vol. 3, no. 6, pp. 1-9, June 2015.
N. S. Nehe and R. S. Holambe, “DWT and LPC based feature extraction methods for isolated word recognition,” EURASIP Journal of Audio, Speech, Music Processing, vol. 2012, no. 1, pp. 1-7, 2012.
T. Kinnunen and H. Li, “An overview of text-independent speaker recognition from features to supervectors,” Speech Communication, vol. 52, no. 1, pp. 12-40, 2010.
F. Zeng and H. Zhou, “Speaker recognition based on a novel hybrid algorithm,” Procedia Engineering, vol. 61, pp. 220-226, 2013.
O. Prabhakar and N. Sahu, “A survey on voice command recognition technique,” International Journal of Advance Research in Computer Science and Software Engineering , vol. 3, no. 5, pp. 576-585, 2013.
M. G. Sumithra, K. Thanuskodi, and A. H. J. Archana, “A new speaker recognition system with combined feature extraction techniques”, Journal of Computer Science, vol. 7, no. 4, pp. 459-465, 2011.
Y. Jeong, “Joint speaker and environment adaptation using tensor voice for robust speech recognition,” Speech Communication, vol. 58, pp. 1-10, 2014.
D. A. Reynolds, T. F. Quatieri, and R. B. Dunn, “Speaker verification using adapted Gaussian mixture models,” Digital Signal Processing, vol. 10, no. 1-3, pp. 19-41, 2000.
L. Moreno, J. G. Dominguez, D. Martinez, O. Plchot, J. G. Rodriguez, and P. Moreno, “On the use of deep feed forward neural networks for automatic language identification,” Computer Speech Language, vol. 40, pp. 46-59, 2016.
R. Price, K. Iso, and K. Shinoda, “Wise teachers train better DNN acoustic models,” EURASIP Journal of Audio, Speech, Music Processing, vol. 2016, no. 1, pp. 1-10, 2016.
T. Alsmadi, H. A. Alissa, E. Trad, and K. A. Alsmadi, “Artificial intelligence for speech recognition based on neural networks”, Journal of Signal and Information Processing, vol. 6, no. 2, pp. 66-72, 2015.
S. Squartini, E. Principi, R. Rotili, and F. Piazza, “Environmental robust speech and speaker recognition through multi-channel histogram equalization,” Neurocomputing, vol. 78, no. 1, pp. 111-120, 2012.
K. Gupta and D. Gupta, “An analysis on LPC, RASTA and MFCC techniques in automatic speech recognition system,” Conf. Cloud System and Big Data Engineering (Confluence), pp. 493-497, 2016.
Z. Li and Y. Gao, “Acoustic feature extraction method for robust speaker identification,” Multimedia Tools and Applications, vol. 75, no. 12, pp. 7391-7406, June 2016.
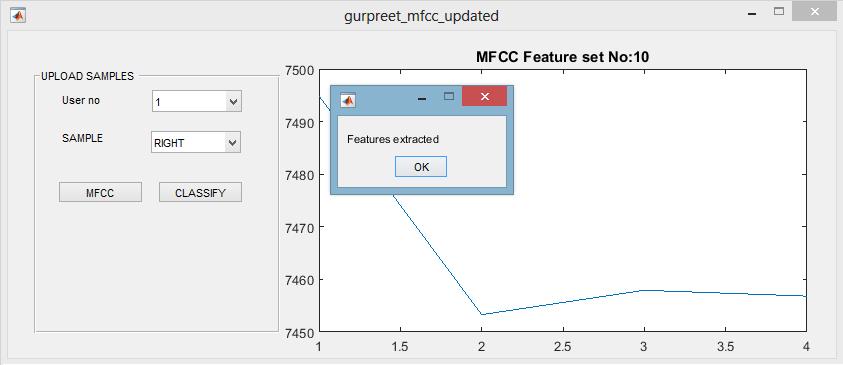
Published
How to Cite
Issue
Section
License
Copyright (c) 2017 International Journal of Engineering and Technology Innovation

This work is licensed under a Creative Commons Attribution-NonCommercial 4.0 International License.
Copyright Notice
Submission of a manuscript implies: that the work described has not been published before that it is not under consideration for publication elsewhere; that if and when the manuscript is accepted for publication. Authors can retain copyright in their articles with no restrictions. Also, author can post the final, peer-reviewed manuscript version (postprint) to any repository or website.
Since Jan. 01, 2019, IJETI will publish new articles with Creative Commons Attribution Non-Commercial License, under Creative Commons Attribution Non-Commercial 4.0 International (CC BY-NC 4.0) License.
The Creative Commons Attribution Non-Commercial (CC-BY-NC) License permits use, distribution and reproduction in any medium, provided the original work is properly cited and is not used for commercial purposes.