A Systematic Review on Automatic Detection of Plasmodium Parasite
DOI:
https://doi.org/10.46604/ijeti.2021.6094Keywords:
systematic literature review, Plasmodium parasite, malaria, machine learingAbstract
Plasmodium parasite is the main cause of malaria which has taken many lives. Some research works have been conducted to detect the Plasmodium parasite automatically. This research aims to identify the development of current research in the area of Plasmodium parasite detection. The research uses a systematic literature review (SLR) approach comprising three stages, namely planning, conducting, and reporting. The search process is based on the keywords which were determined in advance. The selection process involves the inclusion and exclusion criteria. The search yields 45 literatures from five different digital libraries. The identification process finds out that 28 methods are applied and mainly categorizes as machine learning algorithms with performance achievements between 60% and 95%. Overall, the research of Plasmodium parasite detection today has focused on the development with artificial intelligence specifically related to machine and deep learning. These approaches are believed as the most effective approach to detect Plasmodium parasites.
References
F. Yang, M. Poostchi, H. Yu, Z. Zhou, K. Silamut, J. Yu, et al. “Deep Learning for Smartphone-Based Malaria Parasite Detection in Thick Blood Smears,” IEEE Journal of Biomedical and Health Informatics, vol. 24, no. 5, pp. 1427-1438, May 2020.
H. A. Nugroho, W. A. Saputra, A. E. Permanasari, and E. E. H. Murhandarwati, “Automated Determination of Plasmodium Region of Interest on Thin Blood Smear Images,” International Seminar on Intelligent Technology and Its Application (ISITIA), IEEE Press, August 2017, pp. 352-355.
A. R. Bharti, K. P. Patra, R. Chuquiyauri, M. Kosek, R. H. Gilman, A. Llanos-Cuentas, et al., “Short Report: Polymerase Chain Reaction Detection of Plasmodium Vivax and Plasmodium Falciparum DNA from Stored Serum Samples: Implications for Retrospective Diagnosis of Malaria,” The American Journal of Tropical Medicine and Hygiene, vol. 77, no. 3, pp. 444-446, September 2007.
A. S. Abdul Nasir, M. Y. Mashor, and Z. Mohamed, “Segmentation Based Approach for Detection of Malaria Parasites Using Moving K-means Clustering,” IEEE-EMBS Conference on Biomedical Engineering and Sciences, IEEE Press, December 2012, pp. 653-658.
H. A. Nugroho, A. F. D. Marsiano, K. Xaphakdy, P. Sihakhom, E. L. Frannita, R. Nurfauzi, et al. “Multithresholding Approach for Segmenting Plasmodium Parasites,” International Conference on Information Technology and Electrical Engineering (ICITEE), IEEE, October 2019, pp. 1-5.
A. H. Setianingrum, L. K. Wardhani, A. F. Ridwan, and S. F. Nasution, “Identification of Plasmodium Falciparum Stages Using Support Vector Machine Method,” The 7th International Conference on Cyber and IT Service Management (CITSM), IEEE, November 2019, pp. 2-6.
S. Nayak, S. Kumar, and M. Jangid, “Malaria Detection Using Multiple Deep Learning Approaches,” 2nd International Conference on Intelligent Communication and Computational Techniques (ICCT), September 2019, pp. 292-297.
S. S. Prakash, B. C. Kovoor, and K. Visakha, “Convolutional Neural Network Based Malaria Parasite Infection Detection Using Thin Microscopic Blood Smear Samples,” Second International Conference on Inventive Research in Computing Applications (ICIRCA), IEEE, July 2020, pp. 308-313.
G. Shekar, S. Revathy, and E. K. Goud, “Malaria Detection Using Deep Learning,” 4th International Conference on Trends in Electronics and Informatics (ICOEI), IEEE, June 2020, pp. 746-750.
D. Shah, K. Kawale, M. Shah, S. Randive, and R. Mapari, “Malaria Parasite Detection Using Deep Learning: (Beneficial to Humankind),” International Conference on Intelligent Computing and Control Systems (ICICCS), IEEE, May 2020, pp. 984-988.
L. Rosado, J. M. Correia da Costa, D. Elias, and J. S. Cardoso, “A Review of Automatic Malaria Parasites Detection and Segmentation in Microscopic Images,” Journals of Anti-Infective Agents, vol. 14, no. 1, pp. 11-22, March 2016.
Z. Jan, A. Khan, M. Sajjad, K. Muhammad, S. Rho, and I. Mehmood, “A Review on Automated Diagnosis of Malaria Parasite in Microscopic Blood Smears Images,” Journals of Multimedia Tools and Appllications., vol. 77, no. 8, pp. 9801-9826, March 2018.
M. Poostchi, K. Silamut, R. J. Maude, S. Jaeger, and G. Thoma, “Image Analysis and Machine Learning for Detecting Malaria,” Journals of Translational Research., vol. 194, pp. 36-55, April 2018.
R. S. Wahono, “A Systematic Literature Review of Software Defect Prediction: Research Trends, Datasets, Methods and Frameworks,” Journal of Software Engineering, vol. 1, no. 1, pp. 1-16, April 2015.
S. Keele, “Guidelines for Performing Systematic Literature Reviews in Software Engineering,” School of Computer Science and Mathematics, Keele University, EBSE Technical Report-2007-01, July 09, 2007.
R. S. Wahono, “Systematic Literature Review: Pengantar, Tahapan Dan Studi Kasus” https://romisatriawahono.net/2016/05/15/systematic-literature-review-pengantar-tahapan-dan-studi-kasus, April 10, 2020.
P. H. Prastyo, A. S. Sumi, and S. S. Kusumawardani, “A SystematicLliterature Review of Application Development to Realize Paperless Application in Indonesia: Sectors, Platforms, Impacts, and Challenges,” Indonesian Journal of Information System, vol. 2, no. 2, pp. 111-129, February 2020.
E. V. H. S, M. Y. Mashor, A. S. A. Nasir, and H. Jaafar, “A Fast and Accurate Detection of Schizont Plasmodium Falciparum Using Channel Color Space Segmentation Method,” 5th International Conference on Cyber and IT Service Management (CITSM), IEEE, August 2017, pp. 1-4.
H. A. Nugroho, F. L. Tantowi, R. Anggara, TM. D Aldibra, R. Nurfauzi, E. L. Frannita, et al., “Segmentation of Plasmodium Using Saturation Channel of HSV Color Space,” International Conference on Information and Communications Technology (ICOIACT), February 2019, pp. 91-94.
M. M. Aladago, L. Torresani, E. V. Rosca, and A. Malaria, “Semantic Segmentation of The Growth Stages of Plasmodium Parasites Using Convolutional Neural Networks,” IEEE Africon Conference, IEEE, September 2019, pp. 1-7.
A. Pinkaew, T. Limpiti, and A. Trirat, “Automated Classification of Malaria Parasite Species on Thick Blood Film Using Support Vector Machine,” The 2015 Biomedical Engineering International Conference (BMEiCON), IEEE, November 2015.
H. A. Mohammed and I. A. M. Abdelrahman, “Detection and Classification of Malaria in Thin Blood Slide Images,” International Conference on Communication, Control, Computing and Electronics Engineering (ICCCCEE), IEEE, January 2015, pp. 1-5.
W. Kudisthalert, K. Pasupa, and S. Tongsima, “Counting and Classification of Malarial Parasite from Giemsa-stained Thin Film Images,” Journals and Megazines IEEE Access, vol. 8, pp. 78663-78682, April 2020.
M. Elter, E. Hasslmeyer, and T. Zerfass, “Detection of Malaria Parasites in Thick Blood Films,” Annual international conference of the IEEE Engineering in Medicine and Biology Society, IEEE Press, August 2011, pp. 5140-5144.
D. K. Das, M. Ghosh, M. Pal, A. K. Maiti, and C. Chakraborty, “Machine Learning Approach for Automated Screening of Malaria Parasite Using Light Microscopic Images,” Micron, vol. 45, pp. 97-106, February 2013.
S. S. Savkare and S. P. Narote, “Blood Cell Segmentation from Microscopic Blood Images,” International Conference on Information Processing (ICIP), IEEE Press, December 2015, pp. 502-505.
W. Preedanan, M. Phothisonothai, W. Senavongse, and S. Tantisatirapong, “Automated Detection of Plasmodium Falciparum From Giemsa-stained Thin Blood Films,” The 8th International Conference on Knowledge and Smart Technology (KST), IEEE Press, February 2016, pp. 215-218.
Z. Zhang, L. S. Ong, K. Fang, A. Matthew, J. Dauwells. M. Dao, et al., “Image Classification of Unlabeled Malaria Parasites in Red Blood Cells,” The 38th Annual International Conference of the IEEE Engineering in Medicine and Biology Society (EMBS), IEEE Press, August 2016, pp. 3981-3984.
L. Rosado, J. M. C. Da Costa, D. Elias, and J. S. Cardoso, “Automated Detection of Malaria Parasites on Thick Blood Smears Via Mobile Devices,” Procedia Computer Science, vol. 90, pp. 138-144, July 2016.
I. R. Dave, “Image Analysis for Malaria Parasite Detection from Microscopic Images of Thick Blood Smear,” International Conference on Wireless Communications, Signal Processing and Networking (WiSPNET), IEEE Press, March 2017, pp. 1303-1307.
A. R. Prananda, H. A. Nugroho, and I. Ardiyanto, “Enumeration of Plasmodium Parasites on Thin Blood Smear Digital Microscopic Images,”The 5th International Conference on Science in Information Technology (ICSITech), IEEE Press, October 2019, pp. 223-228.
H. A. Nugroho, J. B. Abraham, A. H. Azzira, E. L. Frannita, R. Nurfauzi, F. M. Azif, et al., “Performance of Convolutional Neural Network in Detecting Plasmodium Parasites,” The 9th International Conference on System Engineering and Technology (ICSET), IEEE Press, October 2019, pp. 331-336.
A. Vijayalakshmi and B. Rajesh Kanna, “Deep Learning Approach to Detect Malaria from Microscopic Images,” Journals of Multimedia Tools and Appllications, vol. 79, no. 21, pp. 15297-15317, January 2019.
S. S. Devi, A. Roy, J. Singha, S. A. Sheikh, and R. H. Laskar, “Malaria Infected Erythrocyte Classification Based on A Hybrid Classifier Using Microscopic Images of Thin Blood Smear,” Journals of Multimedia Tools and Appllications, vol. 77, no. 1, pp. 631-660, December 2018.
J. Cervantes, F. Garcia-Lamont, L. Rodríguez-Mazahua, and A. Lopez, “A Comprehensive Survey on Support Vector Machine Classification: Applications, Challenges and Trends,” Journals of Neurocomputing, vol. 408, pp. 189-215, September 2020.
T. K. Bhowmik, P. Ghanty, A. Roy, and S. K. Parui, “SVM-Based Hierarchical Architectures for Handwritten Bangla Character Recognition,” International Journal on Document Analysis and Recognition., vol. 12, no. 2, pp. 97-108, March 2009.
B. Y. Sun, D. S. Huang, and H. T. Fang, “Lidar Signal Denoising Using Least-Squares Support Vector Machine,” IEEE Signal Processing Letters, vol. 12, no. 2, pp. 101-104, February 2005.
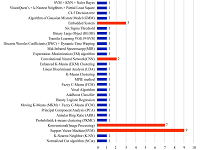
Published
How to Cite
Issue
Section
License
Copyright Notice
Submission of a manuscript implies: that the work described has not been published before that it is not under consideration for publication elsewhere; that if and when the manuscript is accepted for publication. Authors can retain copyright in their articles with no restrictions. Also, author can post the final, peer-reviewed manuscript version (postprint) to any repository or website.
Since Jan. 01, 2019, IJETI will publish new articles with Creative Commons Attribution Non-Commercial License, under Creative Commons Attribution Non-Commercial 4.0 International (CC BY-NC 4.0) License.
The Creative Commons Attribution Non-Commercial (CC-BY-NC) License permits use, distribution and reproduction in any medium, provided the original work is properly cited and is not used for commercial purposes.