Lightweight Compressive Sensing for Joint Compression and Encryption of Sensor Data
DOI:
https://doi.org/10.46604/ijeti.2022.8599Keywords:
sensor data, block compressive sensing, stream cipher, structurally random matrix, pseudo error vectorAbstract
The security and energy efficiency of resource-constrained distributed sensors are the major concerns in the Internet of Things (IoT) network. A novel lightweight compressive sensing (CS) method is proposed in this study for simultaneous compression and encryption of sensor data in IoT scenarios. The proposed method reduces the storage space and transmission cost and increases the IoT security, with joint compression and encryption of data by image sensors. In this proposed method, the cryptographic advantage of CS with a structurally random matrix (SRM) is considered. Block compressive sensing (BCS) with an SRM-based measurement matrix is performed to generate the compressed and primary encrypted data. To enhance security, a stream cipher-based pseudo-error vector is added to corrupt the compressed data, preventing the leakage of statistical information. The experimental results and comparative analyses show that the proposed scheme outperforms the conventional and state-of-art schemes in terms of reconstruction performance and encryption efficiency.
References
T. Alam, “A Reliable Communication Framework and Its Use in Internet of Things (IoT),” International Journal of Scientific Research in Computer Science, Engineering, and Information Technology, vol. 3, no. 5, pp. 450-456, May 2018.
H. M. A. Fahmy, Concepts, Applications, Experimentation and Analysis of Wireless Sensor Networks, 2nd ed., Cham: Springer International Publishing, 2021.
S. A. Jassim and A. K. Farhan, “A Survey on Stream Ciphers for Constrained Environments,” 1st Babylon International Conference on Information Technology and Science, pp. 228-233, August 2021.
D. L. Donoho, “Compressed Sensing,” IEEE Transactions on Information Theory, vol. 52, no. 4, pp. 1289-1306, April 2006.
S. P. Tirani, A. Avokh, and S. Azar, “WDAT-OMS: A Two-Level Scheme for Efficient Data Gathering in Mobile-Sink Wireless Sensor Networks Using Compressive Sensing Theory,” IET Communications, vol. 14, no. 11, pp. 1826-1837, July 2020.
M. Elsisi, M. Q. Tran, K. Mahmoud, D. E. A. Mansour, M. Lehtonen, and M. M. F. Darwish, “Towards Secured Online Monitoring for Digitalized GIS Against Cyber-Attacks Based on IoT and Machine Learning,” IEEE Access, vol. 9, pp. 78415-78427, March 2021.
M. Q. Tran, M. Elsisi, K. Mahmoud, M. K. Liu, M. Lehtonen, and M. M. F. Darwish, “Experimental Setup for Online Fault Diagnosis of Induction Machines via Promising IoT and Machine Learning: Towards Industry 4.0 Empowerment,” IEEE Access, vol. 9, pp. 115429-115441, August 2021.
A. Salim, W. Osamy, A. M. Khedr, A. Aziz, and M. A. Mageed, “A Secure Data Gathering Scheme Based on Properties of Primes and Compressive Sensing for IoT-Based WSNs,” IEEE Sensors Journal, vol. 21, no. 4, pp. 5553-5571, February 2021.
M. Zhang, X. J. Tong, J. Liu, Z. Wang, J. Liu, B. Liu, et al., “Image Compression and Encryption Scheme Based on Compressive Sensing and Fourier Transform,” IEEE Access, vol. 8, pp. 40838-40849, March 2020.
G. Kuldeep and Q. Zhang, “A Novel Efficient Secure and Error-Robust Scheme for Internet of Things Using Compressive Sensing,” IEEE Access, vol. 9, pp. 40903-40914, March 2021.
Y. Zhang, Y. Xiang, L. Y. Zhang, Y. Rong, and S. Guo, “Secure Wireless Communications Based on Compressive Sensing: A Survey,” IEEE Communications Surveys and Tutorials, vol. 21, no. 2, pp. 1093-1111, May 2019.
D. E. Bellasi and L. Benini, “Energy-Efficiency Analysis of Analog and Digital Compressive Sensing in Wireless Sensors,” IEEE Transactions on Circuits and Systems—I: Regular Papers, vol. 62, no. 11, pp. 2718-2729, November 2015.
M. Kaur, D. Singh, V. Kumar, B. B. Gupta, and A. A. A. E. Latif, “Secure and Energy Efficient-Based E-Health Care Framework for Green Internet of Things,” IEEE Transactions on Green Communications and Networking, vol. 5, no. 3, pp. 1223-1231, September 2021.
P. Zhang, S. Wang, K. Guo, and J. Wang, “A Secure Data Collection Scheme Based on Compressive Sensing in Wireless Sensor Networks,” Ad Hoc Networks, vol. 70, pp. 73-84, March 2018.
S. Zhou, Y. He, Y. Liu, C. Li, and J. Zhang, “Multi-Channel Deep Networks for Block-Based Image Compressive Sensing,” IEEE Transactions on Multimedia, vol. 23, pp. 2627-2640, August 2021.
A. S. Unde and P. P. Deepthi, “Block Compressive Sensing: Individual and Joint Reconstruction of Correlated Images,” Journal of Visual Communication and Image Representation, vol. 44, pp. 187-197, April 2017.
A. K. Chatamoni, R. N. Bhukya, and P. R. Jeripotula, “A Novel Approach Based on Compressive Sensing and Fractional Wavelet Transform for Secure Image Transmission,” International Journal of Intelligent Engineering and Systems, vol. 14, no. 4, pp. 11-21, August 2021.
R. Dautov and G. R. Tsouri, “Establishing Secure Measurement Matrix for Compressed Sensing Using Wireless Physical Layer Security,” International Conference on Computing, Networking, and Communications, pp. 354-358, May 2013.
T. T. Do, L. Gan, N. H. Nguyen, and T. D. Tran, “Fast and Efficient Compressive Sensing Using Structurally Random Matrices,” IEEE Transactions on Signal Processing, vol. 60, no. 1, pp. 139-154, January 2012.
“The USC-SIPI Image Database,” http://sipi.usc.edu/database/database.php?volume=misc, 1981.
M. Soos, K. Nohl, and C. Castelluccia, “Extending SAT Solvers to Cryptographic Problems,” International Conference on Theory and Applications of Satisfiability Testing, pp. 244-257, June 2009.
E. J. Candès, J. Romberg, and T. Tao, “Robust Uncertainty Principles: Exact Signal Reconstruction from Highly Incomplete Frequency Information,” IEEE Transactions on Information Theory, vol. 52, no. 2, pp. 489-509, February 2006.
B. M. Alshammari, R. Guesmi, T. Guesmi, H. Alsaif, and A. Alzamil, “Implementing a Symmetric Lightweight Cryptosystem in Highly Constrained IoT Devices by Using a Chaotic S-Box,” Symmetry, vol. 13, no. 1, 129, January 2021.
M. Khan and N. Munir, “A Novel Image Encryption Technique Based on Generalized Advanced Encryption Standard Based on Field of Any Characteristic,” Wireless Personal Communications, vol. 109, no. 2, pp. 849-867, May 2019.
S. Tariq, M. Khan, A. Alghafis, and M. Amin, “A Novel Hybrid Encryption Scheme Based on Chaotic Lorenz System and Logarithmic Key Generation,” Multimedia Tools and Applications, vol. 79, no. 31, pp. 23507-23529, August 2020.
S. Roy, M. Shrivastava, U. Rawat, C. V. Pandey, and S. K. Nayak, “IESCA: An Efficient Image Encryption Scheme Using 2-D Cellular Automata,” Journal of Information Security and Applications, vol. 61, 102919, September 2021.
L. Zhu, H. Song, X. Zhang, M. Yan, T. Zhang, X. Wang, et al., “A Robust Meaningful Image Encryption Scheme Based on Block Compressive Sensing and SVD Embedding,” Signal Processing, vol. 175, 107629, October 2020.
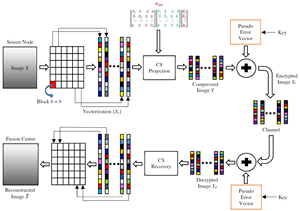
Published
How to Cite
Issue
Section
License
Copyright Notice
Submission of a manuscript implies: that the work described has not been published before that it is not under consideration for publication elsewhere; that if and when the manuscript is accepted for publication. Authors can retain copyright in their articles with no restrictions. Also, author can post the final, peer-reviewed manuscript version (postprint) to any repository or website.
Since Jan. 01, 2019, IJETI will publish new articles with Creative Commons Attribution Non-Commercial License, under Creative Commons Attribution Non-Commercial 4.0 International (CC BY-NC 4.0) License.
The Creative Commons Attribution Non-Commercial (CC-BY-NC) License permits use, distribution and reproduction in any medium, provided the original work is properly cited and is not used for commercial purposes.