Smartwatch/Smartphone Cooperative Indoor Lifelogging System
Keywords:
lifelogging system, smartphone, smartwatch, activity recognitionAbstract
In this study, a lifelogging system is proposed for logging the daily activities of a user using a smartphone and a smartwatch cooperatively in indoor environments. The proposed system attempts to recognize a user’s activities of daily living, including sleeping behavior and various physical activities, and to estimate the user’s daily total energy expenditure (TEE) based on the recognized lifelogs. The TEE has the potential to be useful in personal healthcare management. The system includes both mobile and server systems. The mobile system consists of both a smartwatch and a smartphone used to classify ten activities, including sleeping activities, using sensors on both devices. The server system includes a database server and a set of programs to handle the collected lifelogs for users. An Android app is also developed to display the collected lifelogs and the estimated daily TEE on smartphones to assist in managing users’ health. The experimental results show that the overall average recognition rate of seven activities is 97.5% with four subjects, and the total average error for the three states of sleeping behaviors is 6.64%.References
K. O. Hara, M. M. Tuffield, and N. Shadbolt, “Lifelogging: privacy and empowerment with memories for life,” Identity in the Information Society, vol. 1, pp. 155-172, December 2008.
A. J. Sellen and S. Whittaker, “Beyond total capture: a constructive critique of lifelogging,” Communications of the ACM, vol. 53, no. 5, pp. 70-77, 2010.
R. Rawassizadeh, “Towards sharing life-log information with society,” Behaviour & Information Technology, vol. 31, no. 11, pp. 1057-1067, 2012.
Z. Wang, Z. Yang, and T. Dong, “A review of wearable technologies for elderly care that can accurately track indoor position, recognize physical activities and monitor vital signs in real time,” Sensors, vol. 17, no. 2, pp. 1-36, 2017.
P. Kumar, A. N. Skouloudis, M. Bell, M. Viana, M. C. Carotta, and G. Biskos, “Real-time sensors for indoor air monitoring and challenges ahead in deploying them to urban buildings,” Science of The Total Environment, vol. 560-561, pp. 150-159, August 2016.
R. Rawassizadeh, M. Tomitsch, K. Wac, and A. M. Tjoa, “UbiqLog: a generic mobile phone-based life-log framework,” Personal and Ubiquitous Computing, vol. 17, no. 4, pp. 621-637, April 2013.
P. Belimpasakis, K. Roimela, and Y. You, “Experience explorer: a life-logging platform based on mobile context collection,” Proc. 3rd International Conference on Next Generation Mobile Applications, Services and Technologies, 2009, pp. 77-82.
M. Shoaib, S. Bosch, O. D. Incel, H. Scholten, and P. J. M. Havinga, “Complex human activity recognition using smartphone and wrist-worn motion sensors,” Sensors, vol. 16, pp. 1-24, 2016.
S. Chennuru, P. W. Chen, J. Zhu, and J. Y. Zhang, “Mobile lifelogger - recording, indexing, and understanding a mobile user’s life,” Berlin, Heidelberg, pp. 263-281, 2012.
M. Fahim, I. Fatima, S. Lee, and Y. T. Park, “EFM: evolutionary fuzzy model for dynamic activities recognition using a smartphone accelerometer,” Applied Intelligence, vol. 39, no. 3, pp. 475-488, 2013.
M. Shoaib, H. Scholten, and P. J. M. Havinga, “Towards physical activity recognition using smartphone sensors,” Proc. IEEE 10th International Conference on Ubiquitous Intelligence and Computing and IEEE 10th International Conference on Autonomic and Trusted Computing, 2013, pp. 80-87.
J. W. Lockhart, T. Pulickal, and G. M. Weiss, “Applications of mobile activity recognition,” Proc. 2012 ACM Conference on Ubiquitous Computing, Pittsburgh, Pennsylvania, 2012, pp. 1054-1058.
G. B. Gil, A. Berlanga, and J. M. Molina, “Physical actions architecture: context-aware activity recognition in mobile devices,” Berlin: Heidelberg, pp. 19-27, September 2011.
A. M. Khan, A. Tufail, A. M. Khattak, and T. H. Laine, “Activity recognition on smartphones via sensor-fusion and KDA-based SVMs,” International Journal of Distributed Sensor Networks, vol. 10, no. 5, pp. 1-14, May 2014.
T. S. Kim, J. H. Cho, and J. T. Kim, “Mobile motion sensor-based human activity recognition and energy expenditure estimation in building environments,” Berlin: Heidelberg, pp. 987-993, 2013.
H. Liu, R. Li, S. Liu, S. Tian, and J. Du, “SmartCare: energy-efficient long-term physical activity tracking using smartphones,” Tsinghua Science and Technology, vol. 20, pp. 348-363, August 2015.
H. Jung, Y. D. Lee, and D. U. Jeong, “A novel method for energy expenditure using multisensor based activity monitoring,” Proc. 6th International Conference on Computer Sciences and Convergence Information Technology (ICCIT) , 2011, pp. 103-106.
J. H. Chiang, P. C. Yang, and H. Tu, “Pattern analysis in daily physical activity data for personal health management,” Pervasive and Mobile Computing, vol. 13, pp. 13-25, 2014.
E. A. Chowdhury, M. J. Western, T. E. Nightingale, O. J. Peacock, and D. Thompson, “Assessment of laboratory and daily energy expenditure estimates from consumer multi-sensor physical activity monitors,” PLOS ONE, vol. 12, no. 2, pp. 1-15, February 2017.
M. Duclos, G. Fleury, P. Lacomme, R. Phan, L. Ren, and S. Rousset, “An acceleration vector variance based method for energy expenditure estimation in real-life environment with a smartphone/smartwatch integration,” Expert Systems with Applications, vol. 63, pp. 435-449, November 2016.
“Jawbone,” http://jawbone.com/up, June 20, 2017.
“Fitbit,” http://www.fibit.com/, June 20, 2017.
“Xiaomi wristband,” http://www.mi.com/shouhuan, June 20, 2017.
Z. Chen, M. Lin, F. Chen, N. D. Lane, G. Cardone, R. Wang, and et al., “Unobtrusive sleep monitoring using smartphones,” Proc. 7th International Conference on Pervasive Computing Technologies for Healthcare, Venice, Italy, 2013, pp. 145-152.
W. Gu, Z. Yang, L. Shangguan, W. Sun, K. Jin, and Y. Liu, “Intelligent sleep stage mining service with smartphones,” Proc. 2014 ACM International Joint Conference on Pervasive and Ubiquitous Computing, Seattle, Washington, 2014, pp. 13-17.
D. Picchietti and J. W. Winkelman, “Restless legs syndrome, periodic limb movements in sleep, and depression,” Sleep, vol. 28, no. 7, pp. 891-898, July 2005.
B. Koo, “Periodic limb movements during sleep: review of physiology and pathology,” Journal of Sleep Medicine, vol. 9, no. 9, pp. 23-27, 2012.
N. Nirwal, N. Sardana, and A. J. Bhatt, “Hopeful hearts: a mobile health care application,” Proc. 7th International Conference on Contemporary Computing (IC3), 2014, pp. 351-356.
A. M. Roza and H. M. Shizgal, “The Harris-Benedict equation reevaluated: resting energy requirements and the body cell mass,” The American journal of clinical nutrition, vol. 40, no. 1, pp. 168-182, July 1984.
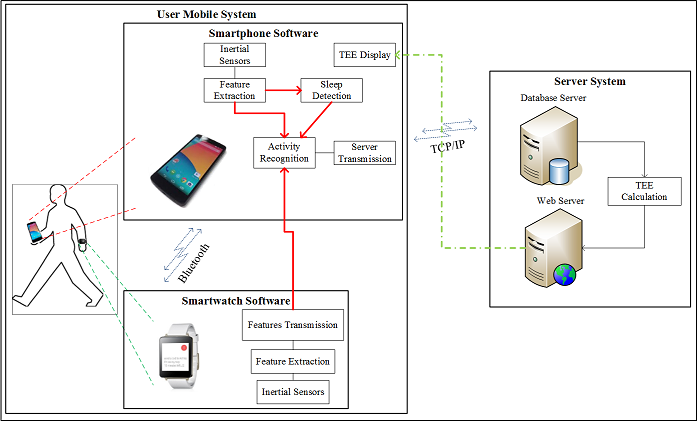
Published
How to Cite
Issue
Section
License
Copyright (c) 2018 International Journal of Engineering and Technology Innovation

This work is licensed under a Creative Commons Attribution-NonCommercial 4.0 International License.
Copyright Notice
Submission of a manuscript implies: that the work described has not been published before that it is not under consideration for publication elsewhere; that if and when the manuscript is accepted for publication. Authors can retain copyright in their articles with no restrictions. Also, author can post the final, peer-reviewed manuscript version (postprint) to any repository or website.
Since Jan. 01, 2019, IJETI will publish new articles with Creative Commons Attribution Non-Commercial License, under Creative Commons Attribution Non-Commercial 4.0 International (CC BY-NC 4.0) License.
The Creative Commons Attribution Non-Commercial (CC-BY-NC) License permits use, distribution and reproduction in any medium, provided the original work is properly cited and is not used for commercial purposes.